Artificial intelligence (AI) is not just a buzzword; it’s a game-changer in the realm of drug discovery. The pharmaceutical industry has long been plagued by lengthy timelines and high costs associated with developing new medications. However, with the advent of AI technologies, we are witnessing a remarkable shift in how drugs are discovered, developed, and brought to market. Imagine a world where the tedious process of sifting through mountains of data is transformed into a streamlined, efficient operation that not only saves time but also enhances the quality of outcomes. This is the promise of AI in drug discovery.
At its core, AI harnesses the power of machine learning and data analytics to predict molecular interactions and identify potential drug candidates at an unprecedented pace. Traditional methods often involve trial and error, which can be both time-consuming and costly. In contrast, AI algorithms can analyse vast datasets, uncovering patterns and insights that may not be immediately apparent to human researchers. This capability allows for the rapid identification of novel compounds and therapeutic targets, effectively accelerating the entire drug discovery pipeline.
Benefits of AI in Drug Discovery | Traditional Methods |
---|---|
Speed: AI can process data and make predictions in a fraction of the time. | Often slow, reliant on manual analysis and experimentation. |
Cost-Effectiveness: Reduces the need for extensive lab trials. | High costs due to lengthy trial phases. |
Precision: More accurate predictions lead to better-targeted therapies. | Less precise, often resulting in trial failures. |
In essence, AI is not merely a tool; it is a catalyst for innovation in pharmaceutical development. As we continue to explore the intersection of technology and medicine, the potential for AI to revolutionise drug discovery becomes increasingly clear. The integration of AI into existing workflows will not only enhance efficiency but also pave the way for breakthroughs that could change the landscape of healthcare as we know it.
As we delve deeper into the capabilities of AI in drug discovery, it’s crucial to acknowledge the challenges that accompany this technological revolution. From data quality to regulatory hurdles, addressing these issues will be key to unlocking the full potential of AI in the pharmaceutical sector.
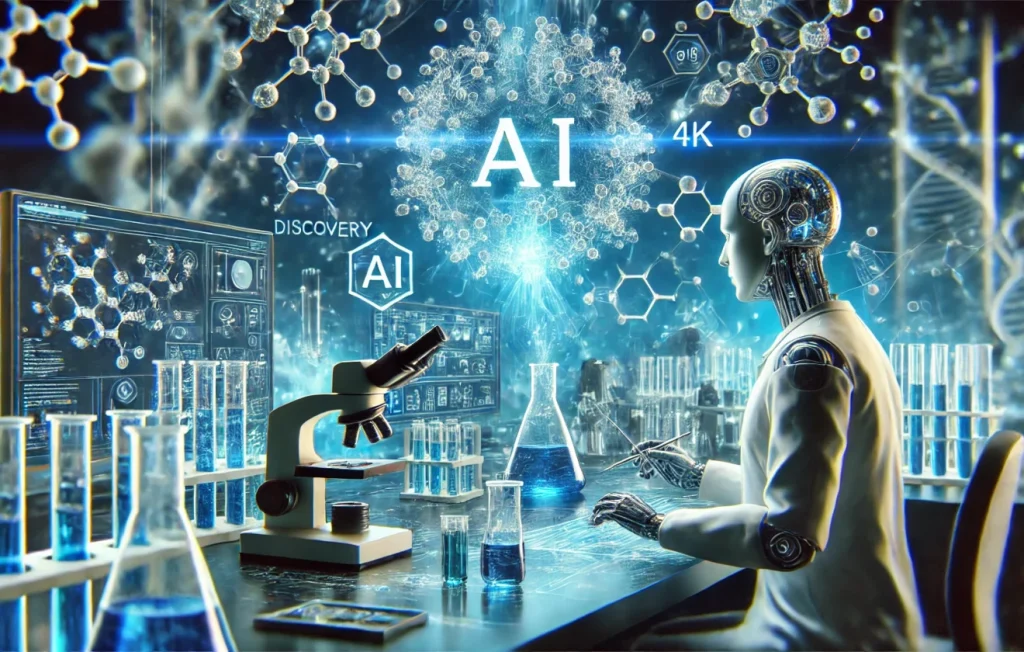
The Role of AI in Drug Discovery
Artificial Intelligence (AI) technologies are fundamentally reshaping the landscape of drug discovery, making it not only faster but also more efficient. Imagine a world where researchers can sift through mountains of data in mere seconds, identifying potential drug candidates that would have taken years to find using traditional methods. This is the reality that AI is crafting, as it streamlines processes and predicts molecular interactions with remarkable accuracy.
At the heart of this transformation lies the ability of AI to analyse vast datasets. By employing machine learning algorithms, researchers can uncover hidden patterns and insights that lead to the discovery of novel compounds and therapeutic targets. It’s akin to having a supercharged detective on your team, tirelessly piecing together clues from complex data sets to unveil solutions that could save lives.
Moreover, AI facilitates predictive modelling, which uses historical data to forecast the success of drug candidates. This significantly reduces the time and costs associated with experimental trials. In fact, studies have shown that AI-driven approaches can cut drug development timelines by up to 50%. That’s a game-changer! However, while the benefits are clear, it’s essential to understand that the integration of AI into drug discovery is not without its challenges.
For instance, the quality and availability of data play a crucial role in the effectiveness of AI models. Without robust data management practices, the outcomes in drug discovery can be unreliable. Additionally, navigating the complex regulatory frameworks can pose hurdles for AI applications, highlighting the need for clear guidelines that foster innovation while ensuring compliance.
In conclusion, the role of AI in drug discovery is pivotal, driving advancements that were once thought impossible. As we continue to harness the power of AI, the pharmaceutical industry stands on the brink of a revolution, promising a future filled with innovative solutions and life-saving drugs.
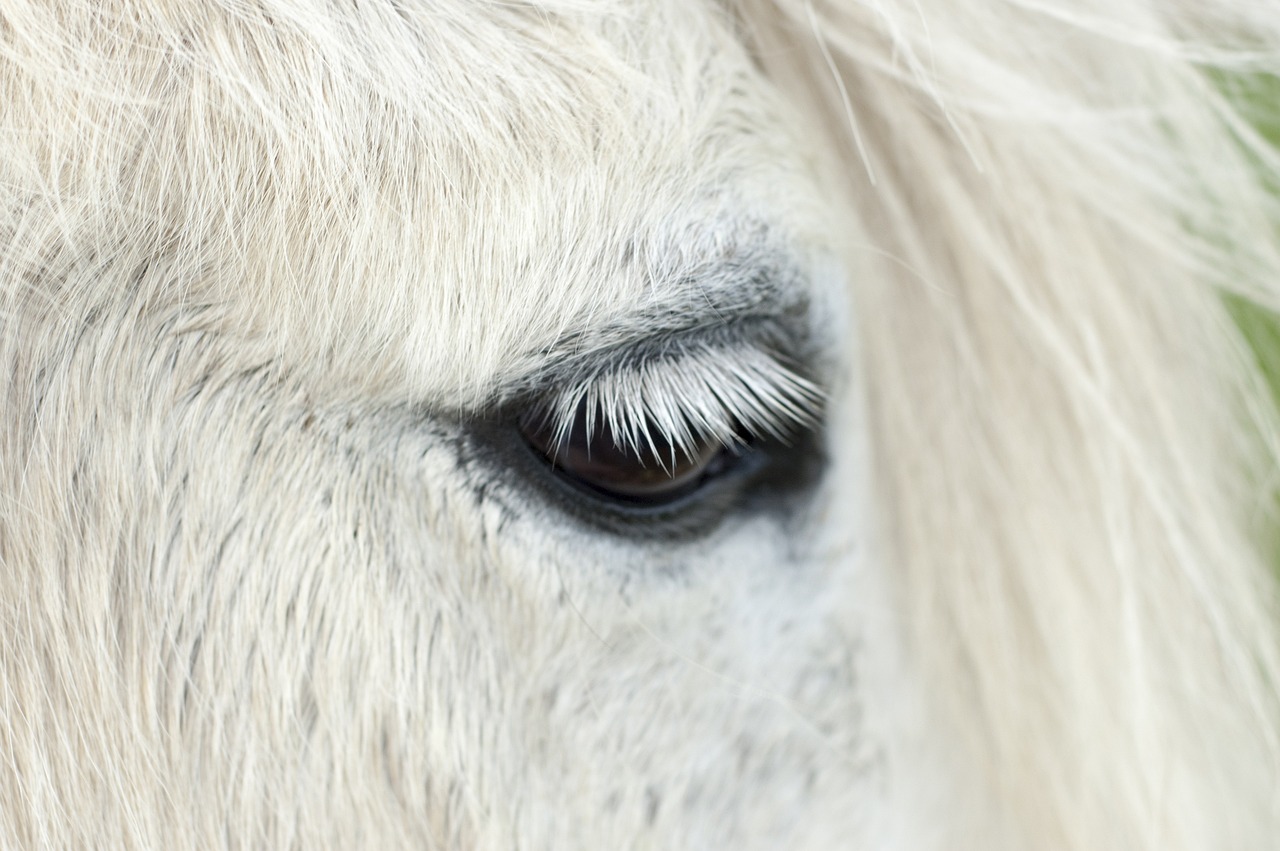
Machine Learning Algorithms in Chemistry
Machine learning algorithms are at the forefront of a **revolution** in the field of chemistry, driving the discovery of novel compounds and therapeutic targets with unprecedented speed and accuracy. By harnessing the power of large datasets, these algorithms can analyse complex chemical interactions and predict outcomes that would take human researchers a significant amount of time to uncover. This transformative technology is not just a tool; it’s a game-changer, reshaping how chemists approach drug discovery.
One of the most exciting aspects of machine learning in chemistry is its ability to identify patterns within vast amounts of data. For instance, algorithms can sift through millions of chemical compounds to find those with the highest potential for success in treating specific diseases. This capability is akin to finding a needle in a haystack, but with the precision that only AI can offer. Moreover, machine learning models can continuously improve as they are fed more data, making them increasingly accurate over time.
To illustrate this further, consider the following table that highlights some of the key machine learning algorithms used in chemistry:
Algorithm | Description | Application |
---|---|---|
Random Forest | A versatile algorithm that uses multiple decision trees to improve prediction accuracy. | Predicting molecular properties. |
Neural Networks | Inspired by the human brain, these models are excellent for recognising complex patterns. | Drug design and optimisation. |
Support Vector Machines | This method finds the best boundary between different classes of data. | Classifying compounds based on activity. |
In addition to these algorithms, researchers are also employing data mining techniques to extract valuable insights from chemical databases. This process allows for the identification of promising drug candidates and optimises lead compounds, making the entire drug discovery pipeline more efficient. However, it is essential to ensure that the data used is of high quality and relevant, as the effectiveness of these algorithms heavily relies on it.
As we delve deeper into the world of machine learning in chemistry, it’s crucial to acknowledge both its potential and the challenges it faces. From data quality issues to the need for regulatory compliance, the journey towards fully integrating AI into drug discovery is ongoing. For further reading on this topic, you can explore resources such as NCBI which provides comprehensive insights into the applications of AI in pharmaceutical development.
Data Mining Techniques
In the realm of drug discovery, have emerged as a beacon of hope, illuminating the path to uncovering valuable insights from vast chemical databases. These techniques are akin to a treasure hunt, where researchers sift through mountains of data to find the hidden gems that could lead to groundbreaking therapeutics. By employing sophisticated algorithms, scientists can identify patterns and correlations that would be nearly impossible to discern through traditional methods.
One of the most impactful aspects of data mining in this field is its ability to facilitate the identification of promising drug candidates. For instance, researchers can utilise machine learning to analyse historical data, which can reveal previously overlooked compounds that might exhibit therapeutic effects. This process not only accelerates the discovery timeline but also enhances the efficiency of resource allocation.
Moreover, data mining techniques can be classified into several categories, each serving a unique purpose in the drug discovery process. These include:
- Classification: This technique categorises compounds based on their properties, helping researchers focus on the most promising candidates.
- Clustering: By grouping similar compounds, clustering can reveal relationships and potential synergies among different molecules.
- Regression Analysis: This method predicts the outcomes of drug interactions, providing valuable insights into efficacy and safety.
As we delve deeper into the data, we must also consider the importance of data quality and availability. The effectiveness of these mining techniques hinges on having access to reliable and comprehensive datasets. Without high-quality data, the insights gained can be misleading or even detrimental to the drug discovery process.
In conclusion, data mining techniques are not just tools; they are essential components of modern drug discovery, enabling researchers to navigate the vast ocean of chemical information and uncover the next wave of pharmaceutical innovations. As these techniques continue to evolve, they hold the promise of transforming how we approach drug development, making it more efficient and effective than ever before.
Predictive Modelling
is a game-changer in the realm of drug discovery, acting as a crystal ball that allows researchers to forecast the success of potential drug candidates. By harnessing the power of historical data, this technique significantly reduces the time and costs associated with experimental trials. Imagine being able to predict which compounds will be effective before even entering the lab—it’s like having a head start in a race!
At its core, predictive modelling employs advanced algorithms that sift through mountains of data to identify patterns and correlations. This process not only enhances the accuracy of predictions but also helps in prioritising candidates that are more likely to succeed in clinical trials. Here are some key aspects of predictive modelling:
- Data-Driven Insights: By analysing past outcomes, predictive models can highlight which characteristics lead to successful drug interactions.
- Cost Efficiency: These models help in saving substantial amounts of money by reducing the number of failed trials.
- Speed: With the ability to rapidly assess multiple candidates, researchers can focus their resources on the most promising options.
Moreover, the integration of machine learning techniques enhances the predictive capabilities of these models. As more data becomes available, the algorithms learn and adapt, continuously improving their accuracy. This iterative learning process is akin to training for a marathon—each run builds endurance for the next.
However, it’s important to note that while predictive modelling offers immense potential, it is not without its challenges. The quality of the data used is paramount; poor data can lead to misleading predictions. Therefore, robust data management practices must be in place to ensure reliable outcomes in drug discovery.
In conclusion, predictive modelling stands at the forefront of pharmaceutical innovation, offering a glimpse into the future of drug development. By embracing this technology, the industry can not only accelerate the discovery process but also pave the way for more effective and targeted therapies. For further reading on this topic, you might find this resource insightful.
Virtual Screening Methods
Virtual screening methods have emerged as a game-changer in the realm of drug discovery, acting as a bridge between computational chemistry and biological targets. These methods allow researchers to simulate interactions between a vast array of compounds and specific biological targets, significantly streamlining the process of identifying potential drug candidates. Imagine having a treasure map that leads you to the most promising compounds without the need to sift through countless options manually!
By employing sophisticated algorithms, virtual screening can efficiently narrow down thousands of compounds to a select few that are most likely to exhibit desired biological activity. This prioritisation not only saves time but also reduces costs associated with experimental testing. The process typically involves several key steps:
- Target Identification: Determining the biological target that the drug will interact with.
- Compound Library Creation: Assembling a diverse library of chemical compounds for screening.
- Docking Studies: Simulating how these compounds bind to the target, predicting their effectiveness.
- Scoring and Ranking: Evaluating the binding affinity to prioritise candidates for further testing.
Moreover, the integration of machine learning techniques enhances the predictive power of virtual screening, allowing for more accurate results. Researchers can continuously refine their models based on feedback from ongoing trials, making this approach not just a one-off process but a dynamic and iterative journey towards drug discovery.
In conclusion, virtual screening methods represent a crucial step in modern drug discovery, enabling faster and more effective identification of potential therapeutics. As the field continues to evolve, the synergy between computational methods and experimental validation will undoubtedly lead to breakthroughs in pharmaceutical development, making the dream of curing diseases a step closer to reality.
AI-Driven Drug Design
is a game-changer in the pharmaceutical industry, revolutionising how new medications are created. By harnessing the power of artificial intelligence, researchers can now design and optimise molecular structures with unprecedented speed and accuracy. Imagine having a super-smart assistant that can sift through mountains of data, find patterns, and suggest the best possible compounds for further testing. This is what AI brings to the table!
One of the most exciting aspects of AI-driven drug design is its ability to tailor molecular properties to meet specific therapeutic needs. For instance, AI algorithms can predict how a drug will interact with biological targets, allowing scientists to create compounds with enhanced efficacy and reduced side effects. This is akin to having a bespoke suit made, where every detail is adjusted to fit perfectly.
Furthermore, the integration of machine learning techniques enables the rapid exploration of vast chemical spaces, dramatically shortening the time from concept to clinical trials. Researchers can employ various approaches, such as:
- Generative models that create novel molecular structures.
- Reinforcement learning to optimise compound properties iteratively.
- Deep learning for predicting biological activity based on chemical structure.
However, despite these advancements, challenges remain. The need for high-quality data is paramount, as the effectiveness of AI models is only as good as the information fed into them. Additionally, regulatory bodies are still catching up with these innovative technologies, which can slow down the implementation of AI-driven processes.
In conclusion, AI-driven drug design is paving the way for a new era of pharmaceutical development, offering the potential to discover and develop drugs faster and more efficiently than ever before. As we continue to embrace these technologies, the future of medicine looks incredibly promising.
AI Techniques | Benefits |
---|---|
Generative Models | Creates novel compounds quickly |
Reinforcement Learning | Optimises properties iteratively |
Deep Learning | Predicts activity from structure |
For more in-depth insights on AI in drug discovery, check out this research article.
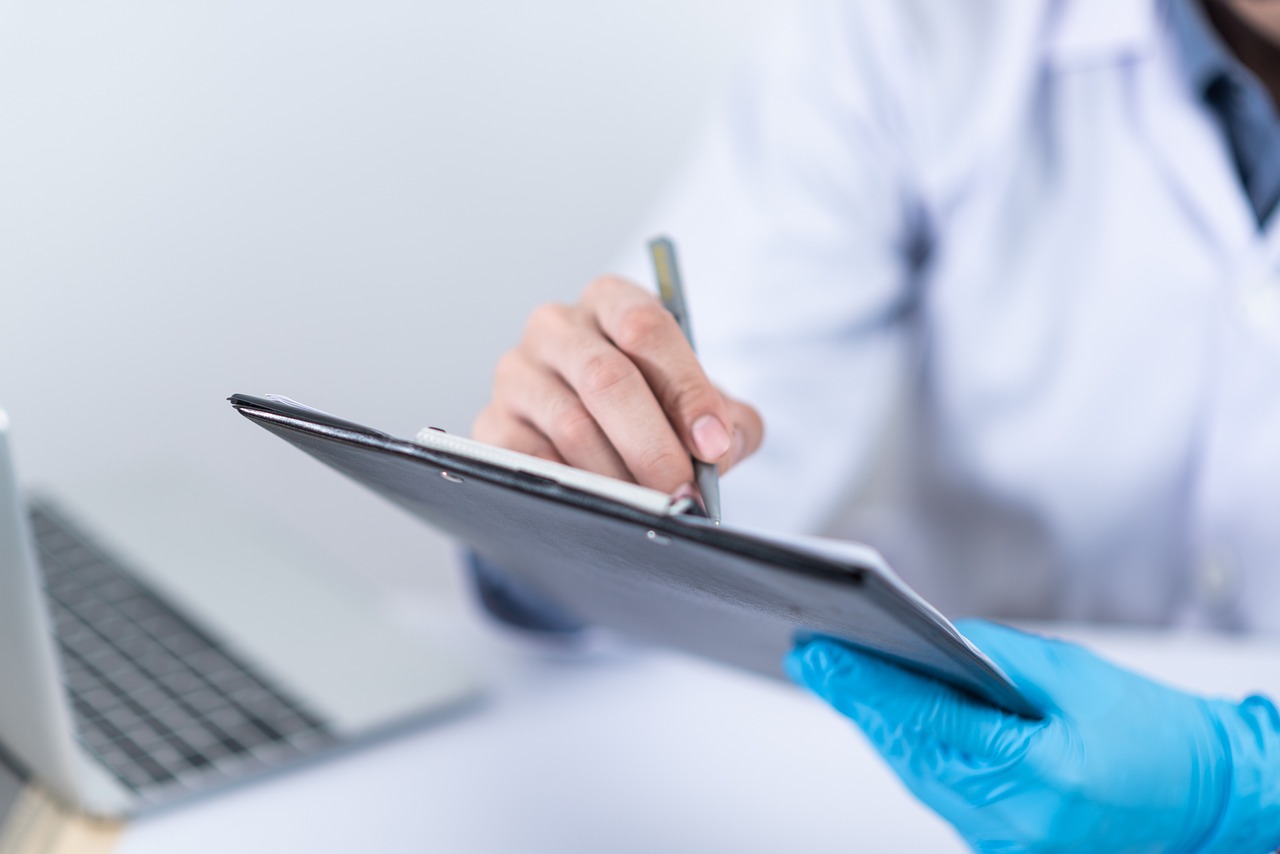
Challenges and Limitations of AI in Drug Discovery
While the integration of artificial intelligence in drug discovery presents exciting opportunities, it is not without its challenges. One of the primary hurdles is the quality and availability of data. AI models thrive on large datasets; however, if the data is incomplete, biased, or poorly structured, the outcomes can be misleading. This necessitates robust data management practices to ensure that the information fed into these systems is both reliable and comprehensive.
Additionally, the interpretability of AI results poses a significant challenge. Researchers often find themselves in a conundrum: while AI can predict outcomes with remarkable accuracy, understanding the rationale behind those predictions can be elusive. This lack of transparency can hinder acceptance among stakeholders who require clear explanations for decision-making.
Moreover, navigating the complex landscape of regulatory hurdles can slow down the adoption of AI technologies in drug discovery. Regulatory frameworks are often not designed with AI in mind, leading to uncertainty about compliance and the need for clear guidelines. This can create a bottleneck, delaying the introduction of innovative solutions into the market.
To illustrate these challenges, consider the following table that summarises the key limitations:
Challenge | Description |
---|---|
Data Quality | Reliance on incomplete or biased datasets undermines AI effectiveness. |
Interpretability | Difficulty in understanding AI predictions can lead to mistrust. |
Regulatory Issues | Complex regulations can impede the integration of AI technologies. |
In conclusion, while AI holds tremendous promise for revolutionising drug discovery, addressing these challenges is essential for its successful implementation. By focusing on improving data quality, enhancing interpretability, and navigating regulatory landscapes, we can unlock the full potential of AI in creating innovative pharmaceutical solutions. For more insights on this topic, check out this article.
Data Quality and Availability
In the realm of AI in drug discovery, the significance of data quality and availability cannot be overstated. The effectiveness of AI models hinges on the robustness of the datasets they are trained on. Imagine trying to build a house on a shaky foundation; it simply won’t hold up. Similarly, if the data fed into these algorithms is flawed or incomplete, the outcomes can be misleading, leading to potential failures in drug development.
Researchers often grapple with issues such as inconsistent data formats, missing values, and outdated information. To combat these challenges, a systematic approach to data management is crucial. This includes:
- Data Curation: Ensuring that datasets are regularly updated and curated to maintain their relevance and accuracy.
- Standardisation: Implementing standard protocols for data entry and storage to facilitate easier analysis.
- Data Integration: Combining data from various sources to create a more comprehensive dataset that enhances the predictive power of AI models.
Moreover, the availability of high-quality data is essential for training machine learning algorithms effectively. As the saying goes, “you can’t make a silk purse out of a sow’s ear.” In this context, if the data is subpar, the results will inevitably reflect that. Therefore, investing in data infrastructure and fostering collaborations between institutions can significantly enhance the landscape of drug discovery.
In summary, prioritising data quality and availability is not just a technical necessity; it’s a strategic imperative. By addressing these issues head-on, the pharmaceutical industry can harness the full potential of AI, leading to faster and more efficient drug discovery processes.
Regulatory Hurdles
When it comes to AI in drug discovery, navigating the complex landscape of regulatory hurdles can feel like trying to find your way through a maze. The pharmaceutical industry is heavily regulated, and introducing AI technologies into this environment raises numerous questions. For instance, how do we ensure that these algorithms comply with existing regulations while still promoting innovation? It’s a delicate balance that requires careful consideration.
One of the primary challenges is the need for clear guidelines that govern the use of AI in drug development. Regulatory bodies are still catching up with the rapid advancements in technology, which means that there is often uncertainty about how AI-driven processes fit into the existing regulatory framework. This uncertainty can lead to delays in the approval of new drugs and therapies, potentially hindering the progress that AI could bring to the field.
Moreover, the reliance on data quality is paramount. Regulatory agencies require robust evidence of safety and efficacy, which means that AI models must be built on high-quality, well-curated datasets. If the data is flawed or biased, it could lead to misleading results, ultimately affecting patient safety. Therefore, ensuring data integrity is not just a technical issue; it’s a regulatory imperative.
To address these challenges, collaboration between regulatory authorities, AI developers, and pharmaceutical companies is essential. Establishing a dialogue can help create standards that foster innovation while ensuring compliance. As the landscape evolves, it’s crucial for all stakeholders to engage in discussions that shape the future of AI in drug discovery.
Challenge | Description |
---|---|
Data Quality | High-quality data is essential for reliable AI outcomes. |
Regulatory Clarity | Clear guidelines are needed to navigate the regulatory landscape. |
Integration Issues | Integrating AI into existing workflows can be complex. |
In conclusion, while the potential for AI to revolutionise drug discovery is vast, addressing these regulatory hurdles is crucial for successful implementation. By fostering a collaborative environment, we can pave the way for a future where AI and pharmaceuticals work hand in hand, ultimately benefiting patients worldwide.