GPT-4 and Beyond: The Evolution of Language Models
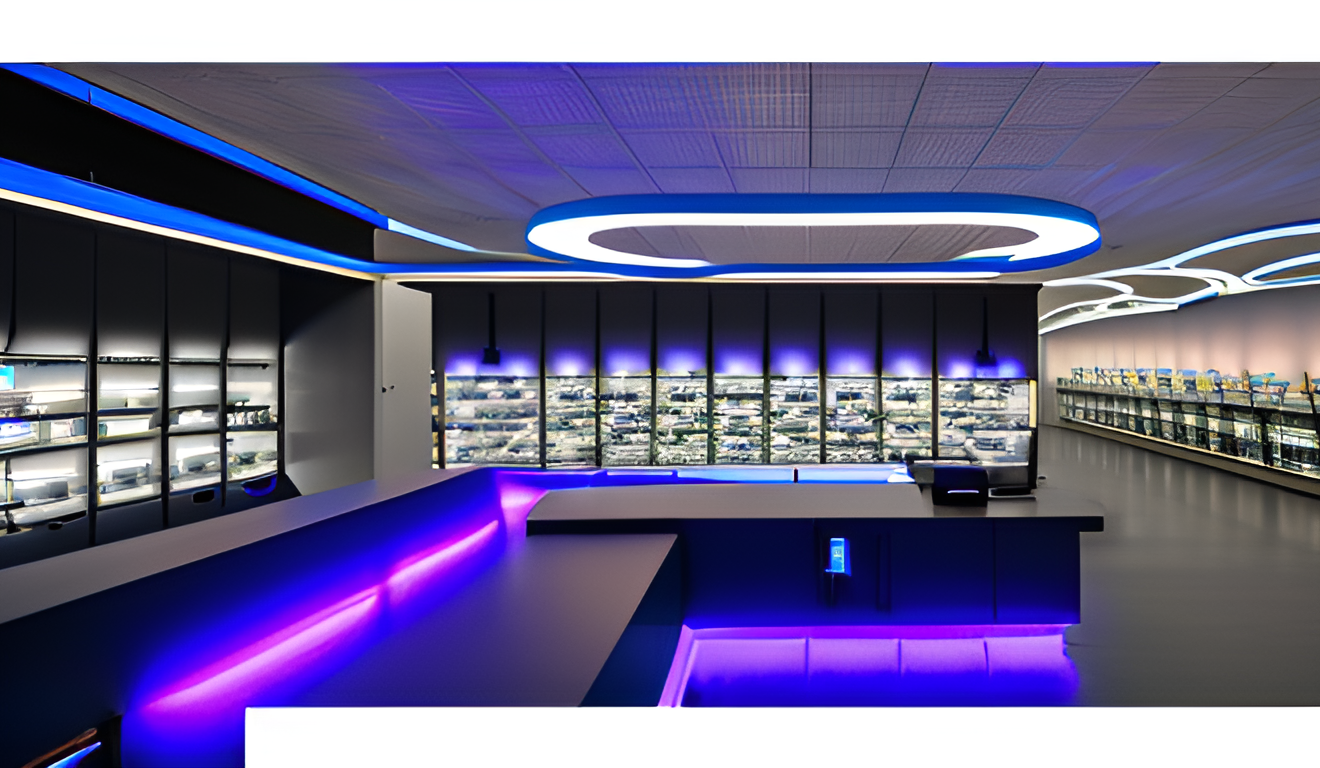
The world of artificial intelligence is witnessing a transformative shift with the advent of advanced language models like GPT-4. These models are not just iterations; they represent a significant leap in how machines understand and generate human language. Imagine a world where computers can converse, write, and even create art just like us—that’s the promise of GPT-4 and its successors.
As we delve into the evolution of these models, it’s essential to recognize the milestones that paved the way for GPT-4. From the early days of simple rule-based systems to the groundbreaking transformer models, the journey has been nothing short of remarkable. Each version has built upon the last, enhancing our ability to communicate with machines in ways that were once thought to be science fiction.
But what exactly sets GPT-4 apart? At its core, the architecture of GPT-4 is designed to mimic human-like text generation with unprecedented accuracy. This is achieved through sophisticated components that allow the model to process vast amounts of information efficiently. The transformer architecture is the backbone of this system, enabling GPT-4 to understand context and generate coherent responses that resonate with users.
As we explore the capabilities of GPT-4, it’s also crucial to consider its applications. From enhancing customer service interactions to revolutionizing content creation in marketing, the potential uses are vast. Businesses can leverage GPT-4 to craft compelling narratives, while developers can utilize it for coding assistance. The implications for various industries are astounding, making it a tool that could redefine productivity and creativity.
However, with great power comes great responsibility. As we embrace these advancements, we must also confront the ethical considerations they bring. Issues such as bias in language generation and the risk of misinformation are critical challenges that need addressing. Ongoing research and responsible usage will be key in ensuring that the benefits of GPT-4 are realized without compromising integrity.
In conclusion, GPT-4 is not just a technological marvel; it represents a new frontier in human-computer interaction. As we stand on the brink of this exciting evolution, the possibilities are limited only by our imagination. What will the future hold for language models? Only time will tell, but one thing is certain: the journey has only just begun.
The Rise of GPT Models
In the ever-evolving landscape of artificial intelligence, the rise of GPT models marks a pivotal moment in our journey toward understanding and generating human-like text. It all began with the introduction of the original GPT, which laid the groundwork for subsequent iterations. With each new version, from GPT-2 to GPT-3, we witnessed a dramatic leap in capabilities. These models not only improved in understanding context but also became adept at generating coherent and contextually relevant responses.
GPT-4, the latest in this lineage, has taken these advancements to new heights. Its architecture is a testament to the rapid progress made in natural language processing (NLP). As we delve deeper into the history of these models, we can identify several key milestones that highlight their significance:
- 2018: The launch of the original GPT model, which introduced the concept of unsupervised learning for language tasks.
- 2019: The arrival of GPT-2, a more powerful version that sparked discussions around the ethical implications of AI-generated content.
- 2020: GPT-3 emerged with a staggering 175 billion parameters, showcasing unprecedented language generation capabilities.
- 2023: GPT-4 debuted, refining its predecessors’ strengths and addressing many limitations, paving the way for broader applications.
These milestones not only illustrate the technological advancements but also highlight the growing significance of GPT models in various fields. As businesses and researchers continue to explore their potential, the implications for industries like marketing, education, and even healthcare are immense. The rise of GPT models is not just a technological trend; it represents a fundamental shift in how we interact with machines and utilize AI to augment human capabilities.
Understanding GPT-4 Architecture
The architecture of GPT-4 represents a significant leap in the evolution of language models, designed to enhance its capabilities in generating human-like text. At its core, GPT-4 utilizes a sophisticated architecture that builds upon the successes of its predecessors while introducing innovative features that elevate its performance. One of the key components of this architecture is the transformer model, which serves as the backbone of GPT-4, enabling it to process and understand language with remarkable efficiency.
Transformers revolutionized natural language processing by allowing models to handle vast amounts of data and learn complex patterns in text. Unlike earlier neural network designs, transformers utilize a self-attention mechanism that enables the model to focus on different parts of the input data dynamically. This means that when GPT-4 reads a sentence, it can weigh the significance of each word relative to others, creating a rich contextual understanding that is crucial for generating coherent and relevant responses.
Transformers are the heart of GPT-4’s architecture, comprising several layers that process information in parallel. Each layer consists of two main components: self-attention and feedforward neural networks. The self-attention mechanism allows the model to evaluate the relationships between words in a sentence, while feedforward networks help in transforming the data into a more refined output. This multi-layered approach not only enhances the model’s ability to generate text but also improves its overall efficiency and speed.
The self-attention mechanism is a game-changer in how GPT-4 interprets language. By analyzing the importance of each word in a given context, the model can generate responses that are not only relevant but also nuanced. For example, in the sentence “The cat sat on the mat,” the model understands that “cat” and “mat” are more closely related than other words, allowing it to create a more coherent narrative.
Another critical aspect of GPT-4’s architecture is the use of layer normalization and feedforward networks. Layer normalization stabilizes the learning process, ensuring that the model remains effective during training. Meanwhile, feedforward networks enhance the model’s capacity to process information, enabling it to generate high-quality text more quickly. Together, these components contribute to GPT-4’s superior performance, making it one of the most advanced language models to date.
Transformers: The Backbone of GPT-4
The advent of transformers has been nothing short of revolutionary in the realm of artificial intelligence, particularly for language models like GPT-4. At its core, the transformer architecture allows for the processing of vast amounts of text data with remarkable efficiency. But what makes it so special? Imagine trying to understand a complex story. You wouldn’t just focus on the first sentence and ignore everything that follows; you’d weave together the entire narrative. That’s precisely what transformers do—they consider the context of words in relation to one another, allowing for a deeper understanding of language.
Transformers utilize a mechanism known as self-attention, which is akin to having a spotlight that shifts focus among different words in a sentence. This enables the model to determine which words carry the most weight in conveying meaning. For example, in the sentence “The cat sat on the mat because it was soft,” the model can discern that “it” refers to “the mat,” enhancing its comprehension. This level of contextual awareness is a game changer, setting GPT-4 apart from its predecessors, which often struggled with maintaining context over longer passages.
Moreover, the architecture is designed with multiple layers, each refining the information processed in the previous layer. This hierarchical approach allows GPT-4 to build intricate representations of language, making it not just a tool for generating text but a sophisticated understanding engine. The combination of layer normalization and feedforward networks within this architecture ensures that the model remains stable and efficient during training and inference, further enhancing its performance.
In summary, transformers are not just a component of GPT-4; they are its backbone. By enabling the model to focus on relevant information and comprehend context at an unprecedented level, transformers have paved the way for the next generation of AI language models. As we continue to explore the capabilities of GPT-4, it’s clear that the transformer architecture is a key player in this evolving landscape of artificial intelligence.
Self-Attention Mechanism
The is a groundbreaking feature of the transformer architecture that powers GPT-4. At its core, this mechanism allows the model to evaluate the significance of each word in a sentence relative to all other words. Imagine reading a sentence and being able to pinpoint which words matter most to grasp the overall meaning. This is precisely what self-attention does, enabling the model to generate contextually relevant responses and understand nuances in language.
When processing a sentence, self-attention assigns different weights to each word based on their relationships. For example, in the sentence “The cat sat on the mat,” the model recognizes that “cat” and “sat” are more closely related than “cat” and “on.” This ability to weigh words dynamically enhances the model’s comprehension and output quality. The self-attention mechanism operates through a series of steps:
- Input Representation: Each word is transformed into a vector representation.
- Score Calculation: The model calculates a score for each word pair, determining how much one word should attend to another.
- Weighting: These scores are normalized to create attention weights, which dictate how much focus to give to each word.
- Output Generation: The weighted representations are combined to produce a final output that reflects the context of the entire sentence.
This process is repeated across multiple layers in the model, allowing for increasingly sophisticated understanding as the data moves through the network. The result? A language model that can generate text that feels remarkably human-like, capturing the subtleties and complexities of natural language in a way that earlier models simply could not. The self-attention mechanism is not just a technical achievement; it represents a significant leap toward making AI more intuitive and effective in understanding and generating language.
Layer Normalization and Feedforward Networks
In the world of deep learning, layer normalization and feedforward networks play crucial roles in enhancing the performance of models like GPT-4. Layer normalization is a technique that helps stabilize the training process by normalizing the inputs across the features for each training example. This means that instead of normalizing across the batch, which can vary in size and composition, layer normalization focuses on the individual example, allowing the model to learn more effectively. Imagine trying to tune a musical instrument; if you only adjust it based on a group performance, it may sound off when played solo. Layer normalization ensures that each piece is in harmony on its own.
On the other hand, feedforward networks are the backbone of the architecture that processes input data in a linear fashion. In GPT-4, after the self-attention mechanism weighs the importance of different words, the information is passed through these feedforward networks. They consist of layers that apply transformations to the data, allowing the model to capture complex relationships and patterns. Think of it as a chef mixing ingredients; each layer adds a unique flavor, contributing to the final dish. The combination of layer normalization and feedforward networks ensures that GPT-4 not only learns quickly but also generalizes well to produce coherent and contextually relevant text.
To illustrate the interaction between these components, consider the following table:
Component | Function |
---|---|
Layer Normalization | Stabilizes training by normalizing inputs across features for each example. |
Feedforward Networks | Processes data in a linear manner, capturing complex relationships. |
In summary, the synergy between layer normalization and feedforward networks is essential for the efficiency and effectiveness of GPT-4. They not only enhance the model’s training but also ensure that it generates human-like text with remarkable fluency and accuracy. As we continue to explore the capabilities of language models, understanding these foundational elements will be key to unlocking their full potential.
Comparative Analysis with Previous Models
The evolution of language models has been nothing short of revolutionary, and GPT-4 stands as a testament to this progress. When we compare GPT-4 to its predecessors, such as GPT-2 and GPT-3, the advancements are striking. Each iteration has brought forth improvements in language understanding, text generation, and overall efficiency. But what exactly sets GPT-4 apart?
One of the most notable differences lies in its ability to generate more coherent and contextually relevant responses. While GPT-3 was already impressive, it occasionally struggled with maintaining context over longer interactions. GPT-4, however, utilizes a more sophisticated architecture, which allows it to retain context more effectively, leading to conversations that feel more natural and engaging.
Moreover, the self-attention mechanism in GPT-4 has been significantly enhanced. This feature enables the model to evaluate the importance of each word in a sentence relative to others, thus improving its understanding of context. For instance, in a conversation about a complex topic, GPT-4 can better grasp nuances and subtleties, providing responses that are not just relevant but insightful.
To illustrate these improvements, consider the following comparison:
Feature | GPT-2 | GPT-3 | GPT-4 |
---|---|---|---|
Context Retention | Limited | Moderate | High |
Response Coherence | Good | Very Good | Excellent |
Understanding Nuance | Poor | Fair | Outstanding |
These enhancements not only improve user experience but also expand the potential applications of GPT-4 across various fields. From creative writing to technical support, the ability to generate high-quality text that closely mimics human conversation opens up a world of possibilities. As we delve deeper into the capabilities of GPT-4, it’s evident that it not only surpasses its predecessors but also sets a new standard for what language models can achieve.
Applications of GPT-4
GPT-4 is not just a leap in technology; it’s a game changer across various fields. Its ability to understand and generate human-like text has opened doors to applications that were once thought to be the stuff of science fiction. Imagine having a virtual assistant that can not only respond to queries but also engage in meaningful conversations. This is the reality that GPT-4 brings to the table, impacting areas such as creative writing, customer service, education, and even programming assistance.
In the realm of content creation and marketing, GPT-4 has become an invaluable tool for marketers. With its advanced capabilities, it can help generate engaging copy, social media posts, and blog articles with remarkable ease and efficiency. Picture a marketer brainstorming ideas; GPT-4 can provide a plethora of suggestions, making the creative process not only faster but also more innovative. This allows brands to maintain a consistent voice while also appealing to diverse audiences.
Moreover, in the field of programming assistance, GPT-4 shines brightly. Developers often face the daunting task of debugging or writing extensive code. With GPT-4, they can receive code suggestions, debugging help, or even entire code snippets generated on-the-fly. This not only streamlines the programming process but also reduces the time spent on mundane tasks, allowing developers to focus on more complex challenges. Consider a developer stuck on a tricky problem; with GPT-4, they can quickly get back on track, much like having a coding buddy available 24/7.
As we explore the applications of GPT-4, it’s evident that this technology is not just about making tasks easier. It is about enhancing creativity, improving efficiency, and transforming the way we interact with machines. Whether you are a marketer looking to craft the perfect message or a programmer seeking a coding partner, GPT-4 is here to revolutionize your experience.
Content Creation and Marketing
In today’s fast-paced digital landscape, content creation and marketing are more crucial than ever. With the rise of platforms that demand fresh, engaging content, businesses are turning to advanced tools like GPT-4 to help them stay ahead of the curve. Imagine having a virtual assistant that can churn out blog posts, social media updates, and marketing copy in a fraction of the time it would take a human. That’s the power of GPT-4!
One of the most exciting aspects of using GPT-4 in content marketing is its ability to generate high-quality, relevant content tailored to specific audiences. By analyzing trends and user preferences, GPT-4 can create compelling narratives that resonate with readers. This not only saves time but also enhances engagement rates, as the content produced is often more aligned with what the audience is looking for.
Furthermore, GPT-4 can assist in various stages of the content creation process. From brainstorming ideas to drafting outlines and even polishing the final product, its capabilities streamline workflows. For instance, marketers can leverage GPT-4 to:
- Generate catchy headlines that grab attention.
- Create detailed blog posts that provide value to readers.
- Draft social media posts that encourage interaction.
By integrating GPT-4 into their strategies, businesses can not only improve efficiency but also enhance their overall brand presence. The AI’s ability to analyze data and understand context means that the content produced is not just filler; it’s strategically crafted to drive results. As we look toward the future, it’s clear that tools like GPT-4 are not just trends—they’re transforming the way we think about content marketing.
Programming Assistance
In today’s fast-paced tech landscape, GPT-4 is emerging as a game-changer for developers seeking programming assistance. Imagine having a smart buddy who not only understands your coding needs but can also provide instant solutions! This is precisely what GPT-4 offers. It helps streamline the coding process, allowing developers to focus on creativity rather than getting bogged down by syntax errors or debugging woes.
One of the most significant advantages of using GPT-4 in programming is its ability to generate code snippets on demand. Whether you’re working on a complex algorithm or just need a quick function, GPT-4 can whip up the necessary code in seconds. For instance, if you’re stuck on a particular task, you can simply describe what you need, and the model will provide a relevant code solution. This not only saves time but also boosts productivity.
Moreover, GPT-4 serves as an excellent debugging partner. When faced with a stubborn bug, developers can describe the issue to the model, and it can suggest potential fixes or point out logical errors. This collaborative approach transforms the debugging process from a frustrating chore into a more manageable task. Think of it as having a seasoned mentor at your side, guiding you through the intricacies of your code.
Another exciting feature of GPT-4 is its capability to assist with documentation. Writing clear and concise documentation is crucial in programming, yet it often gets overlooked. With GPT-4, developers can generate documentation for their code automatically, ensuring that others (and their future selves) can understand the logic behind their work. This not only enhances collaboration but also fosters a culture of clarity and transparency in coding projects.
In conclusion, the integration of GPT-4 into the programming workflow is like adding rocket fuel to your coding engine. By providing instant code generation, debugging support, and documentation assistance, it empowers developers to work smarter, not harder. As we embrace these advancements, the future of programming looks brighter than ever!
Ethical Considerations
As we venture deeper into the realm of advanced language models like GPT-4, it becomes increasingly crucial to address the ethical implications that accompany their deployment. With great power comes great responsibility, and the capabilities of these models raise significant concerns regarding misinformation, bias, and the potential for misuse in various contexts. How do we ensure that the technology serves humanity positively rather than exacerbating existing challenges?
One of the most pressing issues is the emergence of bias in language models. Bias can creep in during the training phase, often reflecting the prejudices present in the data used to train these models. This means that when GPT-4 generates text, it might inadvertently perpetuate stereotypes or provide skewed perspectives. Addressing this issue is not just about improving the model; it involves a commitment to diversity and inclusivity in the datasets and rigorous testing for bias. Developers are actively working on strategies to mitigate these biases, but it remains a complex challenge.
Moreover, the potential for misinformation is another ethical dilemma that cannot be ignored. With the ability to generate coherent and contextually relevant text, GPT-4 could easily be misused to create misleading articles or social media posts that spread false information. This raises the question: how do we balance the benefits of such powerful tools with the risks they pose? It’s essential to implement robust strategies to prevent the spread of misinformation, such as enhancing user awareness and developing guidelines for responsible usage.
In conclusion, as we harness the power of GPT-4 and similar technologies, we must remain vigilant about the ethical landscape. Continuous research, public discourse, and collaboration among developers, ethicists, and users will be vital in navigating these challenges. By prioritizing ethical considerations, we can ensure that advancements in language models contribute positively to society.
Bias in Language Models
As we dive deeper into the world of language models like GPT-4, we can’t ignore a critical issue that looms over their development: bias. Bias in language models refers to the unintended prejudices that can emerge during the training process, often reflecting societal stereotypes or disparities. These biases can manifest in various forms, such as gender, race, or cultural biases, and they can significantly impact the quality and fairness of the generated content.
One key reason for the emergence of bias is the data on which these models are trained. If the training data contains biased information, the model will likely reproduce those biases in its outputs. For instance, if a model is trained predominantly on texts that portray a specific gender in a negative light, it may generate content that perpetuates those stereotypes. This can lead to harmful consequences, especially when such models are deployed in sensitive areas like hiring processes or customer service.
To understand the scope of this issue, consider the following examples of bias that can occur:
- Gender Bias: Models may favor male pronouns in professional contexts, reinforcing stereotypes about gender roles.
- Racial Bias: Language models might generate outputs that are stereotypical or derogatory towards certain racial groups.
- Cultural Bias: Certain cultures may be misrepresented or overlooked entirely in the training data, leading to skewed outputs.
Addressing bias in language models is not just a technical challenge; it’s a societal responsibility. Developers are actively working on strategies to identify and mitigate these biases, such as:
- Implementing diverse training datasets that better represent various demographics.
- Conducting regular audits of model outputs to identify and rectify biased content.
- Incorporating feedback from diverse user groups to improve model performance and fairness.
As we continue to harness the power of AI, it’s crucial to remain vigilant about the ethical implications of these technologies. By understanding and addressing bias, we can strive towards creating language models that are not only powerful but also fair and inclusive.
Preventing Misinformation
In an age where information flows faster than ever, the risk of misinformation becoming a widespread issue is a daunting challenge, especially with advanced language models like GPT-4 at the forefront. As these models generate text that can sometimes mimic human writing with alarming accuracy, it’s crucial to implement strategies that ensure the integrity of the information being disseminated. So, how can we tackle this pressing concern?
First and foremost, responsible usage of AI tools is essential. Users must be educated about the potential pitfalls of relying solely on AI-generated content. This means understanding that while GPT-4 can produce coherent and contextually relevant text, it does not inherently verify the truthfulness of the information. Therefore, it’s vital for users to cross-check facts and consult trusted sources before sharing content that may have been generated by AI.
Moreover, ongoing research into the development of better filtering systems is necessary. By incorporating advanced algorithms that can detect and flag potentially misleading information, developers can create safeguards that help prevent the spread of false narratives. For instance, the integration of fact-checking databases can serve as a reference point for the AI, enhancing its ability to discern credible information from dubious claims.
Additionally, fostering a culture of media literacy among the general public is paramount. When individuals are equipped with the skills to critically evaluate sources and content, they become less susceptible to misinformation. Educational initiatives that focus on teaching people how to identify credible information can significantly reduce the impact of false claims.
In summary, while the capabilities of models like GPT-4 are impressive, they come with a responsibility to ensure that the information shared is accurate and reliable. By promoting responsible usage, investing in filtering technologies, and enhancing media literacy, we can collectively work towards a future where misinformation is minimized, allowing for a more informed society.
Frequently Asked Questions
- What is GPT-4?
GPT-4 is a state-of-the-art language model developed by OpenAI that excels at generating human-like text. It builds on the successes of its predecessors by incorporating advanced architecture and training techniques, making it more capable in understanding and producing language.
- How does GPT-4 differ from previous models?
GPT-4 features improvements in language understanding and generation due to its enhanced transformer architecture, which allows for better context processing and more coherent responses compared to earlier models like GPT-3.
- What are some applications of GPT-4?
GPT-4 is being utilized in various fields, including content creation for marketing, customer service automation, educational tools, and programming assistance, where it helps developers with code suggestions and debugging.
- Are there ethical concerns related to GPT-4?
Yes, the deployment of GPT-4 raises ethical issues, including the potential for misinformation, bias in generated content, and the need for responsible usage to prevent misuse in various contexts.
- How is bias addressed in GPT-4?
Efforts are being made to identify and mitigate biases that can arise during the training of GPT-4. This includes refining training data and implementing techniques to reduce the impact of biased outputs.
- What strategies are in place to prevent misinformation?
To combat misinformation, researchers are focusing on developing guidelines for responsible usage, enhancing model transparency, and encouraging ongoing research to improve the reliability of generated content.