How AI Is Accelerating Particle Physics Research
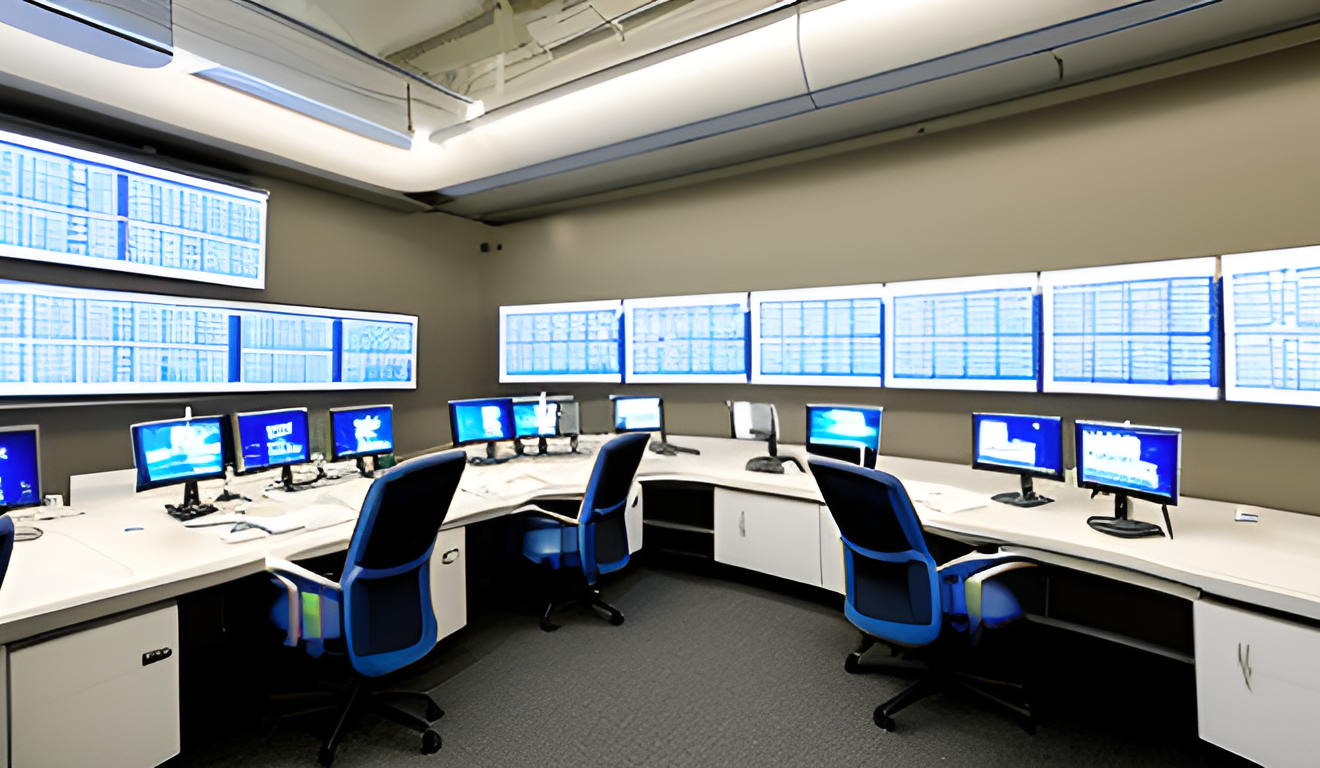
Artificial Intelligence (AI) is not just a buzzword; it’s a game-changer, especially in the realm of particle physics. Imagine standing at the edge of a vast universe of data, where each particle collision generates an overwhelming amount of information. Traditional methods struggle to keep up, but AI swoops in like a superhero, transforming how researchers approach this intricate field. With its ability to analyze data at lightning speed, AI is paving the way for discoveries that could reshape our understanding of the universe.
The integration of AI into particle physics is akin to upgrading from a bicycle to a rocket ship. It allows scientists to harness complex algorithms that can sift through data more efficiently than ever before. For instance, machine learning techniques are being employed to identify patterns in particle collision data—think of it as teaching a computer to recognize faces, but instead, it’s recognizing the unique signatures of subatomic particles. This not only enhances the accuracy of experimental results but also accelerates the pace of research.
Moreover, the use of neural networks in particle detection systems is revolutionizing how particles are classified and identified. These networks mimic the human brain, enabling machines to learn from vast datasets and make predictions with remarkable precision. As a result, physicists can validate their experiments more effectively, ensuring that their findings are robust and reliable.
But it doesn’t stop there. AI is also streamlining the automation of experiments. Imagine a lab where machines adjust parameters in real-time based on incoming data—this is not science fiction; it’s happening now! This automation not only boosts efficiency but also frees up researchers to focus on the creative aspects of their work, fostering an environment ripe for innovation.
In conclusion, the role of AI in particle physics is nothing short of transformative. As we continue to explore the cosmos and delve deeper into the fabric of reality, AI stands as a crucial ally, enhancing our capabilities and opening doors to groundbreaking discoveries that lie ahead.
The Intersection of AI and Particle Physics
Artificial intelligence (AI) is not just a buzzword; it’s a powerful tool that is reshaping the landscape of particle physics. Imagine trying to decode the secrets of the universe with a mountain of data that traditional methods struggle to sift through. This is where AI steps in, acting as a sophisticated guide through the labyrinth of information. By integrating AI into particle physics, researchers are discovering innovative methodologies that enhance their understanding of fundamental particles and their interactions.
At its core, the intersection of AI and particle physics is about leveraging advanced algorithms to tackle complex problems. For instance, AI systems can analyze vast datasets generated by particle accelerators, which produce millions of collision events every second. Traditional data analysis methods can be slow and cumbersome, but AI algorithms can process this information in a fraction of the time. This efficiency not only accelerates the discovery process but also opens up new avenues for exploration that were previously unimaginable.
One of the most exciting aspects of this integration is the ability to identify patterns and anomalies in data. AI models can recognize subtle signals that may indicate new physics beyond the Standard Model. This capability is akin to finding a needle in a haystack, where the needle represents a groundbreaking discovery waiting to be made. With AI’s help, physicists can focus their efforts on the most promising areas of research, significantly increasing the likelihood of significant breakthroughs.
Furthermore, the collaboration between AI and particle physics is paving the way for enhanced experimental designs. By simulating various scenarios using AI, researchers can optimize their experiments before they even begin. This proactive approach not only saves time and resources but also ensures that experiments are set up to yield the most informative results. In essence, AI is transforming the way physicists think about and conduct their research, making the impossible possible.
Data Analysis Revolutionized
In the realm of particle physics, the explosion of data generated by experiments is both a blessing and a challenge. Traditional data analysis methods often struggle to keep pace with the sheer volume of information, making it difficult for researchers to extract meaningful insights. Enter artificial intelligence (AI), a game-changer that is revolutionizing the way scientists analyze data. By harnessing the power of AI algorithms, physicists can now sift through vast datasets with unprecedented speed and accuracy.
Imagine trying to find a needle in a haystack. Now, picture having a super-intelligent robot that can not only find that needle but also analyze its properties and predict its behavior. That’s the kind of transformation AI brings to particle physics data analysis. With machine learning techniques at the forefront, researchers are able to identify patterns and correlations that would have previously gone unnoticed.
A significant aspect of this revolution is the application of machine learning techniques. These methods, including both supervised and unsupervised learning, allow researchers to train algorithms on existing datasets. As a result, these algorithms become adept at recognizing complex patterns in particle collision data. This leads to enhanced accuracy in experimental results and predictions, ultimately accelerating the pace of discovery.
Utilizing machine learning in particle physics is akin to having a personal assistant who learns your preferences over time. The more data the algorithms process, the better they become at making predictions. For instance, when analyzing collision events, AI can help physicists pinpoint the most significant interactions, filtering out the noise and focusing on what truly matters. This capability not only streamlines the analysis process but also enhances the reliability of the results.
Moreover, neural networks have emerged as a crucial tool in particle detection systems. These networks are designed to classify and identify subatomic particles with remarkable precision. By mimicking the way human brains process information, neural networks can differentiate between various particle types, which is essential for validating experimental outcomes. The ability to accurately classify particles leads to more reliable data, paving the way for groundbreaking discoveries.
In conclusion, the integration of AI into data analysis is transforming particle physics in ways that were once thought impossible. With enhanced efficiency and accuracy, researchers are now equipped to tackle the complexities of particle interactions more effectively, opening up new avenues for exploration and understanding in this fascinating field.
Machine Learning Techniques
In the rapidly evolving world of particle physics, are proving to be game-changers. These methods, which include both supervised and unsupervised learning, enable researchers to sift through massive datasets generated by particle collisions. Imagine trying to find a needle in a haystack, where the haystack is a mountain of data—this is where machine learning shines. By applying these advanced algorithms, scientists can identify patterns and anomalies that would be nearly impossible to detect manually.
Supervised learning, for instance, involves training a model on a labeled dataset, allowing it to learn from examples. Once trained, the model can then predict outcomes for new, unseen data. This technique is particularly useful in particle physics for tasks such as event classification, where researchers need to categorize collision events based on their characteristics. On the other hand, unsupervised learning does not rely on labeled data. Instead, it identifies inherent structures within the data, making it invaluable for discovering new phenomena or unexpected behaviors in particle interactions.
One of the most exciting applications of these techniques is in the realm of data classification. For example, researchers can use machine learning to analyze collision data from particle accelerators like the Large Hadron Collider (LHC). By feeding these algorithms vast amounts of data, they can learn to distinguish between different types of particles and interactions. This capability not only enhances the accuracy of experimental results but also accelerates the pace of discovery.
To illustrate, consider the following table that summarizes the key differences between supervised and unsupervised learning:
Technique | Definition | Application in Particle Physics |
---|---|---|
Supervised Learning | Learning from labeled data to make predictions. | Event classification based on known particle types. |
Unsupervised Learning | Identifying patterns in unlabeled data. | Discovering new particle interactions or anomalies. |
In conclusion, the integration of machine learning techniques into particle physics research is not just a trend; it’s a fundamental shift that is enhancing our understanding of the universe. As these technologies continue to evolve, they promise to unlock new avenues of discovery, making the field more exciting than ever.
Neural Networks in Particle Detection
Neural networks are becoming a game changer in the field of particle detection, acting like a digital brain that processes and interprets complex data. Imagine trying to find a needle in a haystack; now, think of how neural networks can sift through vast amounts of collision data to pinpoint subatomic particles with remarkable precision. These advanced algorithms mimic the way human brains work, learning from vast datasets and improving their accuracy over time. This capability is crucial, as the identification and classification of particles are essential for verifying experimental results.
One of the most exciting aspects of using neural networks in particle detection is their ability to enhance the speed and efficiency of data analysis. Traditional methods often struggle with the sheer volume of data generated by particle collisions, leading to bottlenecks in research. However, neural networks can process this information in real-time, allowing physicists to make swift decisions and adjustments during experiments. For instance, when a particle collision occurs, the neural network can rapidly analyze the resulting data to determine the types of particles produced, as well as their trajectories.
Moreover, the flexibility of neural networks means they can be trained for various tasks within particle detection. Some common applications include:
- Classifying different types of particles based on their energy levels.
- Identifying rare particle events that might indicate new physics.
- Predicting the outcomes of collisions before they happen, which can save time and resources in experimental setups.
As researchers continue to refine these systems, the potential for discovering new particles and understanding fundamental forces increases exponentially. The integration of neural networks into particle detection not only enhances the accuracy of experiments but also opens up new avenues for exploration in the realm of particle physics. With every advancement, we get closer to unlocking the mysteries of the universe, one particle at a time.
Predictive Modeling
In the rapidly evolving realm of particle physics, is emerging as a game changer. Using advanced AI algorithms, physicists can now forecast the outcomes of particle collisions with remarkable precision. Imagine trying to predict the weather; just like meteorologists analyze patterns to forecast rain or sunshine, researchers in particle physics use predictive modeling to anticipate the results of complex experiments. This capability not only enhances the accuracy of their predictions but also saves valuable time and resources.
One of the most significant advantages of AI-driven predictive modeling is its ability to guide experimental setups. Before a physicist even steps into the lab, they can simulate various collision scenarios and optimize their approach based on the predicted outcomes. This is akin to a chef perfecting a recipe before cooking; by tweaking ingredients based on expected flavors, they can create a masterpiece. In the same way, predictive modeling allows physicists to refine their experiments before they even begin.
Moreover, predictive modeling facilitates better resource allocation in large-scale physics experiments. With vast amounts of data generated from particle collisions, determining where to focus efforts can be overwhelming. However, AI can analyze historical data and trends, helping researchers prioritize certain experiments that are more likely to yield significant results. This strategic approach is crucial in a field where time and funding are often limited.
To illustrate the impact of predictive modeling, consider the following table that summarizes its key benefits:
Benefit | Description |
---|---|
Enhanced Accuracy | Improves the precision of experimental predictions, leading to more reliable results. |
Resource Optimization | Helps allocate resources efficiently by identifying high-potential experiments. |
Time Efficiency | Reduces the time spent on experiments by allowing for pre-experiment simulations. |
In conclusion, as predictive modeling continues to evolve, it promises to unlock new avenues of exploration in particle physics. By harnessing the power of AI, researchers are not just reacting to data; they are proactively shaping the future of their experiments. This shift from reactive to predictive approaches is akin to moving from a rearview mirror to a GPS system—enabling physicists to navigate the complexities of particle interactions with unprecedented clarity and foresight.
Automation of Experiments
The realm of particle physics is undergoing a significant transformation thanks to the . By leveraging the power of artificial intelligence, researchers can now streamline complex processes that were once time-consuming and prone to human error. Imagine a world where experiments can run themselves, adjusting in real-time based on incoming data. This is not just a dream; it’s becoming a reality!
Automation in particle physics allows for real-time adjustments during experiments, which is crucial for maximizing data collection efficiency. For instance, AI systems can monitor various parameters, such as temperature and pressure, and make necessary adjustments without human intervention. This leads to a more controlled environment, ensuring that the data collected is both accurate and reliable.
One of the key benefits of automating experiments is the ability to handle vast amounts of data. Traditional methods often struggle with the sheer volume of information generated during particle collisions. However, AI can process this data at lightning speed, enabling researchers to uncover patterns and insights that would otherwise remain hidden.
Moreover, the integration of AI in automation allows physicists to focus on the creative aspects of their work rather than getting bogged down by repetitive tasks. This shift not only enhances productivity but also fosters innovation, as scientists have more time to explore new ideas and hypotheses.
In summary, the automation of experiments in particle physics is not just about efficiency; it’s about opening new frontiers in research. As AI continues to evolve, we can expect even greater advancements that will help us unravel the mysteries of the universe. The future looks bright, and the possibilities are endless!
Enhancing Simulation Capabilities
Artificial intelligence is not just a buzzword; it’s a game-changer in the realm of particle physics, particularly when it comes to enhancing simulation capabilities. Imagine trying to predict the behavior of particles that are smaller than atoms—it’s like trying to forecast the weather in a place you’ve never been to. AI steps in here as a powerful ally, creating more accurate models of particle interactions that help physicists explore theoretical scenarios that were once deemed impractical.
One of the standout innovations in this area is the use of Generative Models, particularly Generative Adversarial Networks (GANs). These models are like talented artists, capable of generating new data that mimics real-world particle interactions. By simulating complex scenarios, GANs provide invaluable insights that can aid in hypothesis testing and experimental design. For instance, researchers can test various conditions and see how particles might behave without the need for costly and time-consuming experiments.
Moreover, AI-driven real-time feedback systems are revolutionizing the experimental landscape. Picture this: physicists conducting an experiment and receiving instant feedback on their data. This capability allows for immediate adjustments, enhancing the overall quality of the experiments. The result? Improved outcomes and a faster path to groundbreaking discoveries. With AI at the helm, the traditional barriers of time and resource limitations are being dismantled, paving the way for more ambitious projects in particle physics.
As we look to the future, the integration of AI in simulation capabilities is expected to grow even more robust. The synergy between AI and particle physics not only accelerates the pace of research but also opens doors to new questions and explorations that could redefine our understanding of the universe. It’s an exciting time for physicists, as they harness the power of AI to push the boundaries of what’s possible in their field.
Generative Models
Generative models, particularly Generative Adversarial Networks (GANs), are making waves in the field of particle physics. These sophisticated algorithms enable researchers to simulate complex particle interactions that were once deemed too intricate to model accurately. Imagine trying to predict the outcome of a cosmic event with countless variables at play; that’s where generative models shine, providing a way to visualize and interpret these chaotic phenomena.
At their core, generative models work by learning the underlying distributions of data from existing datasets. This means they can create new, synthetic data that mirrors real-world scenarios, which is invaluable for hypothesis testing and experimental design. For instance, when physicists are exploring new theories about particle behavior, they can use these models to generate potential outcomes of particle collisions. This not only saves time but also enhances the robustness of their experiments.
Here’s how generative models contribute to particle physics research:
- Enhanced Data Generation: They create high-quality synthetic datasets that help researchers test their theories without the need for extensive real-world experiments.
- Improved Hypothesis Testing: By simulating various scenarios, scientists can evaluate the validity of their hypotheses more efficiently.
- Resource Optimization: Generative models allow for better allocation of resources in large-scale experiments by predicting which tests are likely to yield the most significant insights.
As we look to the future, the integration of generative models in particle physics is only expected to grow. With continuous advancements in AI technology, these models will likely become even more sophisticated, providing deeper insights into the fundamental workings of the universe. In essence, generative models are not just tools; they are transformative agents that are reshaping how we understand particle interactions and paving the way for groundbreaking discoveries in physics.
Real-Time Feedback Systems
In the fast-paced world of particle physics, are proving to be game-changers. Imagine being in a race where every second counts, and instead of waiting for the finish line to see how you performed, you get instant updates on your speed and technique. That’s exactly what these systems do for physicists. They provide immediate insights into ongoing experiments, allowing researchers to make on-the-fly adjustments that can significantly enhance the quality of their data.
These systems utilize advanced algorithms to analyze incoming data streams from experiments, enabling scientists to detect anomalies or unexpected results almost instantly. This capability is crucial in particle physics, where minor fluctuations can lead to major discoveries or, conversely, significant errors in data interpretation. By harnessing the power of artificial intelligence, researchers can quickly identify when something isn’t going according to plan and take corrective action before it’s too late.
One of the most exciting aspects of real-time feedback systems is their ability to facilitate adaptive experimentation. This means that as data is collected, the parameters of the experiment can be adjusted to optimize outcomes. For instance, if a particular particle interaction isn’t yielding the expected results, the system can suggest modifications to the experimental setup, such as changing the collision energy or adjusting the detector configuration. This level of adaptability not only saves time but also maximizes the potential for groundbreaking discoveries.
Moreover, the integration of these systems with machine learning techniques allows for even more sophisticated analysis. As the system learns from past experiments, it becomes better at predicting which adjustments are likely to yield the best results. This creates a feedback loop that continuously improves the efficiency and effectiveness of particle physics research. The future of experimentation is not just about collecting data; it’s about making that data work harder and smarter for scientists.
Future Prospects of AI in Physics
The future of artificial intelligence in the realm of particle physics is not just bright; it’s positively dazzling! As technology continues to evolve at a breakneck pace, the integration of AI is expected to revolutionize how physicists approach research and experimentation. Imagine a world where complex calculations that once took weeks can be completed in mere minutes, all thanks to the power of AI. This is not a distant dream but a rapidly approaching reality.
One of the most exciting prospects is the potential for enhanced collaboration between AI systems and human researchers. With AI handling the heavy lifting of data analysis and simulation, scientists can focus more on creative problem-solving and theoretical exploration. This synergy could lead to breakthroughs that redefine our understanding of the universe. For instance, AI might uncover new particles or interactions that eluded detection through traditional methods.
Moreover, advancements in machine learning algorithms will likely enable AI to adapt and learn from experimental results in real time. This adaptability means that researchers could modify their experiments on-the-fly, optimizing conditions to yield the most informative data. Picture a particle collider where AI not only predicts outcomes but also suggests adjustments to maximize discovery potential!
As we look ahead, the implications of AI in particle physics are profound. Researchers are already exploring the use of quantum computing in conjunction with AI, which could further enhance computational power and efficiency. This combination could unlock new frontiers in physics that we have yet to imagine. The table below summarizes some key areas where AI is expected to make a significant impact:
Area of Impact | Potential Benefits |
---|---|
Data Analysis | Faster processing of experimental data |
Experiment Automation | Real-time adjustments to experiments |
Predictive Modeling | Improved forecasting of particle interactions |
Collaboration | Enhanced synergy between AI and researchers |
In conclusion, the future of AI in particle physics is not just about efficiency; it’s about redefining the very fabric of scientific discovery. As we continue to embrace these technologies, who knows what astonishing revelations await us? The universe is vast and full of mysteries, and with AI as our ally, we are poised to uncover them like never before.
Frequently Asked Questions
- How is AI changing particle physics research?
AI is revolutionizing particle physics by enhancing data analysis, automating experiments, and improving simulation capabilities. With machine learning and neural networks, researchers can process vast amounts of data more efficiently, leading to quicker discoveries and more accurate results.
- What role do machine learning techniques play in particle physics?
Machine learning techniques, such as supervised and unsupervised learning, are essential for identifying patterns in particle collision data. These techniques help physicists enhance the accuracy of their experimental results and predictions, ultimately leading to better understanding of subatomic particles.
- What are generative models and how are they used?
Generative models, like GANs (Generative Adversarial Networks), simulate complex particle interactions. They provide valuable insights for hypothesis testing and experimental design, allowing researchers to explore scenarios that were previously impractical.
- Can AI help in real-time data analysis during experiments?
Absolutely! AI-driven real-time feedback systems allow physicists to make immediate adjustments based on incoming data, which significantly improves the outcomes of experiments. This capability is a game-changer in the fast-paced world of particle physics research.
- What does the future hold for AI in particle physics?
The future is bright! Ongoing advancements in AI technology are expected to further enhance research capabilities in particle physics, potentially leading to groundbreaking discoveries in fundamental physics. The integration of AI will continue to push the boundaries of what we can achieve in this complex field.