How AI Predicts Natural Disasters with Greater Accuracy
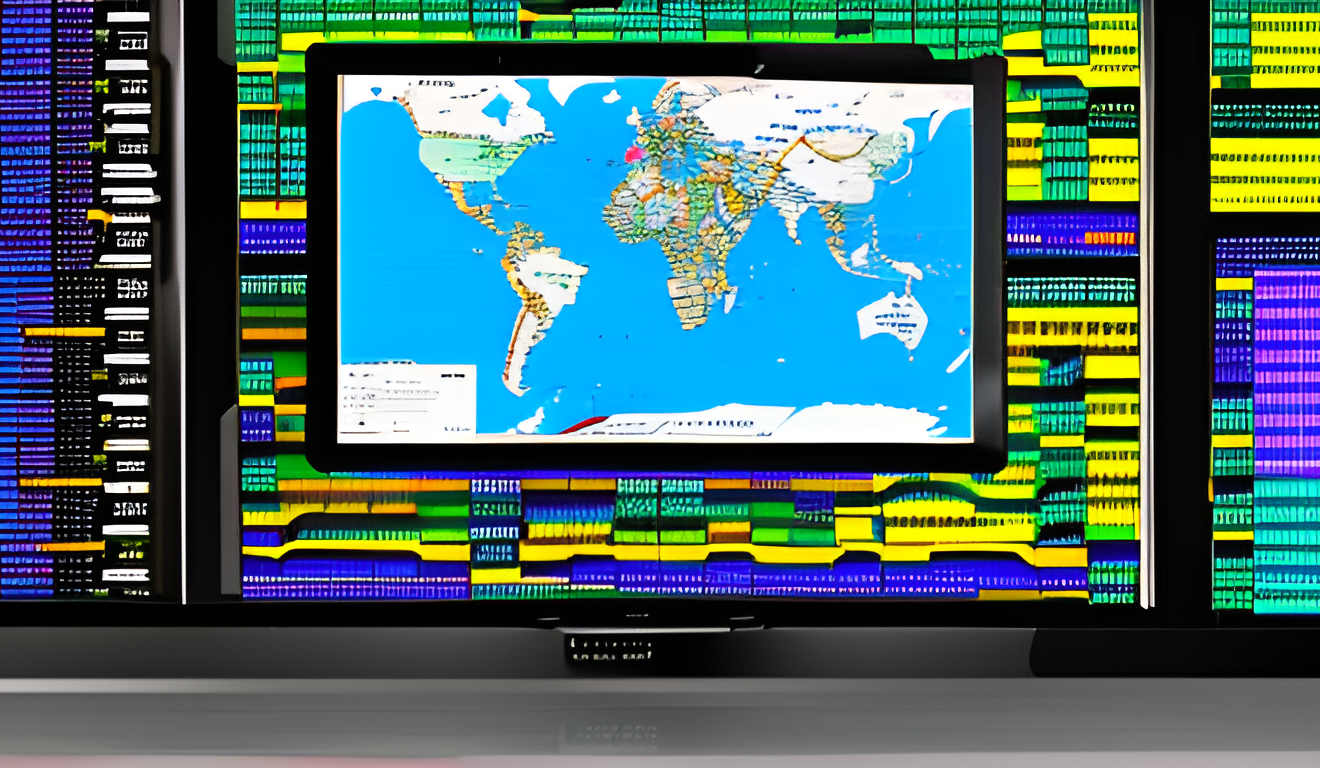
In recent years, artificial intelligence (AI) has emerged as a game changer in the realm of natural disaster prediction and management. Imagine a world where we can anticipate the fury of a hurricane or the tremors of an earthquake before they strike. AI is making this a reality by harnessing the power of data analysis and machine learning to uncover patterns that traditional methods often miss. This technology is not just about crunching numbers; it’s about saving lives and minimizing damage. By integrating AI into our disaster response strategies, we’re not only enhancing our predictive capabilities but also paving the way for more effective preparedness and recovery efforts.
AI technologies have revolutionized how we predict natural disasters, using data analysis and machine learning to identify patterns and forecast events more accurately than traditional methods. Unlike conventional systems that rely on static models, AI continuously learns and adapts, providing a dynamic approach to disaster prediction. This means that as new data comes in, AI systems get smarter, yielding insights that can lead to quicker and more effective responses.
The effectiveness of AI in disaster prediction relies heavily on the quality and variety of data sources. These include:
- Satellite Imagery: Provides critical visual data for monitoring environmental changes.
- Seismic Data: Essential for predicting earthquakes and volcanic activities.
- Meteorological Information: Vital for forecasting storms and floods.
By combining these diverse data streams, AI can create a comprehensive picture of potential disaster scenarios, allowing for timely and informed decision-making.
Satellite imagery plays a crucial role in monitoring environmental changes and disaster indicators. AI algorithms analyze vast amounts of visual data to detect early warning signs, which can be vital for timely predictions and alerts. For instance, by examining changes in land temperature or vegetation health, AI can signal the likelihood of wildfires or drought.
AI systems can analyze real-time satellite data to detect early signs of disasters, enabling quicker responses and potentially saving lives. The ability to provide timely warnings to affected populations is one of the most significant advantages of AI in disaster prediction.
By examining historical disaster data, AI can identify trends and predict future occurrences. This capability makes AI an invaluable tool for disaster preparedness and risk mitigation strategies, allowing communities to better allocate resources and prepare for potential threats.
Machine learning algorithms are at the heart of AI’s predictive capabilities. These systems learn from past data and improve prediction accuracy over time through continuous feedback and adaptation. This iterative process not only enhances the reliability of predictions but also builds a more resilient framework for disaster response.
The Role of AI in Disaster Prediction
Artificial Intelligence (AI) is reshaping the landscape of disaster prediction like never before. Imagine a world where we can foresee natural calamities with astonishing accuracy—this is not just a dream but a reality driven by cutting-edge AI technologies. By harnessing the power of data analysis and machine learning, AI has transformed how we understand and predict disasters, allowing us to identify patterns that were previously invisible to the human eye.
Gone are the days when disaster prediction relied solely on outdated methods and gut feelings. Today, AI systems analyze vast amounts of data from various sources to deliver timely forecasts. For instance, these systems can sift through historical data, weather patterns, and even social media feeds to detect early warning signs of impending disasters. This multi-faceted approach not only enhances prediction accuracy but also equips emergency responders with the information they need to act swiftly.
To illustrate the effectiveness of AI in this domain, consider the following:
- Pattern Recognition: AI algorithms excel at recognizing complex patterns in data that humans might overlook. This capability is crucial for predicting events such as earthquakes or floods.
- Real-time Analysis: AI can process and analyze data in real-time, allowing for immediate alerts and warnings when disaster indicators are detected.
- Continuous Learning: Machine learning models improve over time, refining their predictions as they learn from new data and past events.
Ultimately, the role of AI in disaster prediction is not just about technology; it’s about saving lives. By providing accurate forecasts and timely alerts, AI empowers communities to prepare better and respond more effectively to natural disasters. As we continue to advance in this field, the potential for AI to enhance our disaster management capabilities seems limitless.
Data Sources for AI Predictions
When it comes to predicting natural disasters, the effectiveness of artificial intelligence (AI) is largely dependent on the quality and variety of data it utilizes. AI systems thrive on data, and the more diverse the sources, the more accurate the predictions. Key data sources include satellite imagery, seismic data, and meteorological information. Each of these plays a vital role in providing critical insights that drive accurate forecasting.
For instance, satellite imagery serves as the eyes in the sky, capturing real-time changes in the environment. This visual data is crucial for AI algorithms, enabling them to analyze vast swathes of information quickly and efficiently. Imagine trying to find a needle in a haystack; now imagine having a powerful magnet that pulls out all the needles at once. That’s what satellite imagery does for disaster prediction—it highlights potential threats before they escalate.
With the advent of advanced remote sensing technologies, AI can monitor environmental changes at an unprecedented scale. These systems can detect early signs of disasters, such as rising water levels or unusual seismic activity, which are critical for timely interventions. In fact, AI can process this data in real-time, allowing for quicker responses that can save lives. The ability to provide alerts to affected populations could mean the difference between safety and catastrophe.
Real-time monitoring is a game-changer. By continuously analyzing satellite data, AI systems can detect anomalies that might indicate an impending disaster. For example, if an AI detects a sudden increase in temperature in a forested area, it can trigger alerts for potential wildfires. This rapid detection capability is akin to having a smoke detector in your home—early warnings can lead to lifesaving actions.
In addition to real-time data, AI leverages historical data to identify trends and predict future occurrences. By examining past disasters, AI can recognize patterns that might not be immediately obvious to human analysts. This predictive power is invaluable for developing disaster preparedness and risk mitigation strategies. Think of it like a weather forecast; understanding past weather patterns helps meteorologists predict future storms.
In summary, the integration of diverse data sources is paramount for AI to enhance its predictive capabilities in disaster management. By combining satellite imagery, seismic data, and historical analysis, AI becomes a powerful ally in the fight against natural disasters, paving the way for a safer future.
Satellite Imagery and Remote Sensing
Satellite imagery is a game-changer in the realm of disaster prediction. It provides us with a bird’s-eye view of our planet, capturing vast amounts of data that can reveal crucial environmental changes. Imagine having the ability to see the world from above, detecting subtle shifts in land use, vegetation health, and even water levels. This visual data is not just pretty pictures; it is vital for monitoring disaster indicators such as floods, wildfires, and hurricanes.
AI algorithms thrive on this data, processing it to identify patterns that might be invisible to the naked eye. For instance, by analyzing satellite images over time, AI can spot the early signs of a potential disaster. This capability is akin to having a weathered sailor who can read the ocean’s mood; they know when a storm is brewing long before it hits the shore. By utilizing remote sensing technology, we can detect changes in temperature, moisture levels, and even atmospheric conditions, all of which are critical for accurate disaster forecasting.
One of the most impressive features of AI-driven satellite imagery is its real-time monitoring capabilities. As natural disasters unfold, AI systems can analyze incoming data almost instantaneously, allowing for rapid response. For example, during a hurricane, satellite imagery can provide updates on the storm’s path and intensity, enabling authorities to issue timely warnings to at-risk communities. This quick action can mean the difference between life and death, making satellite data an indispensable tool in disaster management.
Moreover, the integration of historical satellite imagery with current data enhances predictive accuracy. By examining past events and their corresponding satellite images, AI can learn from historical trends and improve its forecasting abilities. This dynamic process ensures that as new data comes in, the algorithms adapt and refine their predictions, creating a feedback loop that continuously enhances our understanding of potential disasters.
Real-time Monitoring Capabilities
The advent of powered by artificial intelligence has transformed the landscape of disaster prediction. Imagine a world where we can detect the early signs of a natural disaster before it strikes, saving countless lives and resources. AI systems utilize real-time satellite data to continuously analyze environmental conditions, looking for anomalies that could indicate an impending disaster. This proactive approach is akin to having a weather radar that not only forecasts storms but also alerts us to their formation as they happen.
One of the most significant advantages of these capabilities is the ability to provide timely warnings to affected populations. For instance, when AI algorithms detect unusual seismic activity or rapidly changing weather patterns, they can trigger alerts that reach local authorities and emergency services within minutes. This swift action can be the difference between life and death. In fact, studies have shown that early warnings can reduce the impact of disasters by up to 30%.
Moreover, AI’s real-time monitoring extends beyond just immediate alerts. It also facilitates ongoing assessments of disaster impacts, allowing responders to adapt their strategies on the fly. For example, during a wildfire, AI can monitor changes in fire behavior and wind patterns, enabling firefighters to adjust their tactics and resources accordingly. This dynamic response capability is crucial in managing complex disasters where conditions can change rapidly.
To illustrate the effectiveness of AI in real-time monitoring, consider the following table:
Disaster Type | AI Monitoring Techniques | Response Time Improvement |
---|---|---|
Earthquakes | Seismic data analysis | Up to 5 minutes |
Hurricanes | Satellite imagery and weather data | 24-48 hours |
Floods | Real-time river level monitoring | Up to 6 hours |
In conclusion, the integration of real-time monitoring capabilities through AI not only enhances our ability to predict natural disasters but also empowers communities to respond more effectively. With every technological advancement, we move closer to a future where disasters can be managed with greater precision and efficiency, ultimately leading to safer environments for all.
Historical Data Analysis
When it comes to predicting natural disasters, plays a pivotal role. Imagine trying to navigate a stormy sea without knowing the weather patterns of the past; it would be nearly impossible. Similarly, AI leverages historical data to uncover trends that help forecast future disasters. By examining past events, AI can identify recurring patterns and anomalies that signal potential risks.
For instance, let’s consider the analysis of hurricane data. By studying the frequency, intensity, and paths of hurricanes over the years, AI systems can predict the likelihood of future storms and their potential impact on specific regions. This predictive capability is not just about looking at numbers; it’s about understanding the story they tell. Each data point is like a breadcrumb leading us to a clearer picture of what might happen next.
The process involves several key steps:
- Data Collection: Gathering comprehensive datasets from various sources, including weather stations, satellite imagery, and historical records.
- Pattern Recognition: Utilizing machine learning algorithms to analyze the collected data for trends and correlations.
- Model Development: Creating predictive models that simulate potential disaster scenarios based on the identified patterns.
- Validation: Testing the models against real-world events to ensure accuracy and reliability.
Moreover, the integration of geospatial analysis allows AI to visualize historical data geographically. This spatial context is crucial for understanding how disasters have impacted different areas over time. By mapping out past events, emergency planners can better prepare for future occurrences, tailoring their response strategies to the specific vulnerabilities of each location.
In summary, the analysis of historical data is not just a technical process; it’s a vital tool that empowers AI to enhance disaster preparedness. As we continue to refine these methods, the potential for saving lives and reducing damage becomes increasingly promising.
Machine Learning Algorithms
At the core of AI’s ability to predict natural disasters are . These algorithms are like the brains of the operation, constantly learning and evolving from the data they process. Imagine a sponge soaking up information; that’s how these algorithms work. They analyze vast amounts of historical and real-time data, identifying patterns that humans might miss. For instance, they can sift through years of seismic activity to find subtle signals that precede an earthquake. This ability to learn from past events and adapt to new information makes them incredibly powerful.
One of the most exciting aspects of machine learning in disaster prediction is its capacity for continuous improvement. As more data becomes available, these algorithms refine their predictions, becoming more accurate over time. This is akin to a seasoned detective who, with each case solved, becomes better at piecing together clues. By employing techniques such as supervised learning, where algorithms are trained on labeled datasets, and unsupervised learning, which identifies patterns without pre-existing labels, machine learning can enhance its predictive capabilities significantly.
Additionally, machine learning can be categorized into various types, each serving a unique purpose in disaster prediction:
- Classification Algorithms: These algorithms categorize data into predefined classes, helping to identify whether conditions are likely to lead to a disaster.
- Regression Algorithms: Used to predict continuous outcomes, these algorithms can forecast the magnitude of a potential natural disaster.
- Clustering Algorithms: They group similar data points, which can help in identifying hotspots for potential disasters.
However, it’s essential to remember that while machine learning algorithms are powerful, they are not infallible. Their effectiveness hinges on the quality of the data fed into them. Inaccurate or biased data can lead to flawed predictions, which could have dire consequences during disaster management. Thus, ensuring data integrity and implementing robust validation processes are critical to harnessing the full potential of these algorithms.
Benefits of AI in Disaster Management
The integration of AI in disaster management is a game-changer, offering a plethora of advantages that significantly enhance our ability to predict, respond to, and recover from natural disasters. Imagine having a tool that can not only foresee potential disasters but also streamline the entire management process. That’s exactly what AI brings to the table! With its ability to analyze vast amounts of data, AI can improve prediction accuracy, resulting in timely alerts that can save countless lives.
One of the most remarkable benefits of AI is its capacity for enhanced decision-making. During a disaster, the stakes are incredibly high, and quick, informed decisions are crucial. AI systems provide data-driven insights that help decision-makers prioritize resources effectively. For instance, they can analyze real-time data to determine which areas are most at risk, allowing for targeted interventions. This not only optimizes response strategies but also ensures that aid reaches those who need it most.
Furthermore, the cost-effectiveness of utilizing AI in disaster management cannot be overstated. By minimizing damage through timely interventions, AI significantly reduces the financial burden associated with disaster response and recovery. For example, a study revealed that communities using AI predictive models saw a reduction in disaster-related costs by up to 30%. This is a win-win situation: saving lives while also conserving resources.
In addition, AI enhances resource allocation by analyzing trends and patterns from previous disasters. This allows organizations to prepare better and allocate resources more efficiently. With AI, we can create a more resilient future, where communities are better equipped to handle the unexpected.
In conclusion, the benefits of AI in disaster management are vast and transformative. From improved prediction accuracy to cost savings and enhanced decision-making, AI is revolutionizing how we prepare for and respond to natural disasters. The future of disaster management is here, and it’s powered by artificial intelligence!
Enhanced Decision-Making
In the chaotic aftermath of a natural disaster, decision-making can often feel like trying to navigate a ship through a storm without a compass. This is where artificial intelligence (AI) steps in as a powerful ally, transforming how emergency responders and government officials make critical choices. By harnessing vast amounts of data, AI provides insights that are not only timely but also incredibly accurate, allowing for a more strategic approach to disaster management.
One of the most significant advantages of AI in decision-making is its ability to analyze data from multiple sources in real-time. For instance, during a hurricane, AI can process information from weather satellites, social media feeds, and local sensors to paint a comprehensive picture of the situation. This means that decision-makers can prioritize resources and formulate strategies based on the most current information available. Imagine having a tool that can sift through mountains of data and highlight the most critical factors—this is the reality that AI brings to the table.
Moreover, AI algorithms can simulate various scenarios, allowing officials to see potential outcomes based on different courses of action. This predictive modeling is invaluable; it empowers leaders to choose paths that maximize safety and efficiency. For example, if a flood is imminent, AI can help determine the best evacuation routes by analyzing traffic patterns and predicting where congestion might occur.
The integration of AI into decision-making processes also enhances collaboration among various agencies. With a centralized system that provides a clear view of the situation, different organizations can coordinate their efforts more effectively. This is crucial during disasters when every second counts, and miscommunication can lead to dire consequences.
In summary, AI is not just a tool; it is a game-changer in disaster management. By enhancing decision-making capabilities, it allows for quicker, more informed responses that can save lives and reduce damage. The future of disaster response is undoubtedly intertwined with the advancements in AI technology, making it an essential component of any effective emergency management strategy.
Cost-Effectiveness
When it comes to disaster management, the of AI technologies is nothing short of revolutionary. Imagine being able to save millions of dollars while also saving lives; that’s the promise AI brings to the table. By leveraging advanced algorithms and data analytics, AI can predict disasters more accurately, allowing for timely interventions that significantly reduce the financial burden on governments and organizations.
For instance, consider the costs associated with a natural disaster. These can include emergency response, recovery efforts, and rebuilding infrastructure. With AI, the ability to predict disasters can lead to proactive measures instead of reactive responses. This means that resources can be allocated more efficiently, focusing on areas that are most at risk. In fact, studies have shown that every dollar spent on disaster preparedness can save up to $6 in recovery costs.
Furthermore, AI enhances resource allocation by identifying the most effective strategies for disaster response. This not only minimizes waste but also ensures that aid reaches those in need more swiftly. For example, during a hurricane, AI can analyze real-time data to determine which areas are likely to be hardest hit, allowing for targeted evacuations and distribution of supplies.
In summary, the of AI in disaster management isn’t just about saving money; it’s about saving lives and ensuring a quicker recovery. By investing in AI technologies, we can create a more resilient future, where communities are better prepared for the unexpected. The future of disaster management looks bright, and AI is leading the charge!
Challenges and Limitations of AI Predictions
While the integration of artificial intelligence in predicting natural disasters has shown remarkable potential, it is not without its challenges and limitations. One of the primary hurdles is the quality and availability of data. AI systems rely heavily on vast datasets to make accurate predictions. However, if these datasets are incomplete or contain inaccuracies, the resulting predictions can be flawed. For instance, if satellite imagery is not updated regularly or if seismic data is sparse, AI algorithms may misinterpret critical signals that indicate an impending disaster.
Moreover, algorithm biases can pose significant challenges. If the data used to train AI models reflects historical biases or inaccuracies, the predictions made by these systems can perpetuate those issues. This can lead to inequitable responses during disasters, where certain populations may receive less attention or resources based on skewed data interpretations. Ensuring that AI systems are trained on diverse and representative datasets is crucial to mitigate this risk.
Another critical limitation is the need for continuous updates to AI algorithms. Natural disasters are influenced by a multitude of factors that can change rapidly. Therefore, AI systems must be regularly calibrated and updated to incorporate new data and insights. Without ongoing adjustments, there is a risk that predictions may become outdated, leading to inadequate responses during actual events.
Lastly, the ethical considerations surrounding AI in disaster prediction cannot be overlooked. As these technologies become more prevalent, issues related to data privacy, algorithm transparency, and accountability emerge. It is essential for developers and organizations to prioritize ethical standards to ensure that AI is used responsibly and maintains public trust. Addressing these challenges head-on will be vital for the successful implementation of AI in disaster management.
Data Quality and Availability
The effectiveness of AI predictions in the realm of natural disasters is heavily dependent on the quality and availability of the data it utilizes. Imagine trying to build a house without the right materials; similarly, AI systems require accurate and comprehensive datasets to function optimally. If the data is incomplete or flawed, the predictions generated can lead to disastrous consequences, leaving communities unprepared when they need help the most.
For instance, consider the various types of data that AI relies on for disaster prediction:
- Seismic Data: Essential for predicting earthquakes, this data must be precise and gathered from reliable sources.
- Meteorological Information: Weather patterns can change rapidly, so having up-to-date information is crucial for forecasting storms and floods.
- Satellite Imagery: Provides visual evidence of environmental changes, but it must be processed accurately to yield meaningful insights.
Moreover, the availability of this data can vary significantly from one region to another. In some areas, advanced technology allows for real-time data collection, while in others, outdated systems can hinder effective monitoring. This disparity can create significant gaps in disaster readiness, making it vital for governments and organizations to invest in improving data infrastructure.
To illustrate the importance of data quality, consider the following table that highlights the impact of data reliability on AI predictions:
Data Quality Level | Prediction Accuracy | Response Effectiveness |
---|---|---|
High Quality | 90%+ | Very Effective |
Moderate Quality | 70%-89% | Effective |
Low Quality | Below 70% | Ineffective |
In conclusion, without a solid foundation of high-quality data, AI’s potential to predict natural disasters accurately is severely compromised. As we advance in technology, it is crucial to address these data challenges to ensure that AI can truly be a game-changer in disaster management.
Ethical Considerations
As the integration of AI technologies into disaster prediction systems becomes more prevalent, it raises several that must be addressed. One of the primary concerns is data privacy. The collection and analysis of vast amounts of data, which often include sensitive information about individuals and communities, necessitate stringent measures to protect personal privacy. If mishandled, this data could lead to breaches of privacy and trust, which is the last thing we want when communities are already vulnerable during disasters.
Another critical issue is algorithmic transparency. Many AI systems operate as “black boxes,” meaning their internal workings are not easily understood, even by their creators. This lack of transparency can lead to algorithmic biases, where certain groups may be unfairly prioritized or neglected in disaster response efforts. For example, if an AI model is trained predominantly on data from urban areas, it might fail to predict disasters impacting rural communities accurately.
Moreover, the responsibility for decisions made by AI systems is still a gray area. Who is accountable when an AI system makes a wrong prediction that results in loss of life or property? This question of accountability is essential as we move forward with AI in disaster management. Stakeholders must ensure that there are clear guidelines and frameworks in place that define responsibilities and facilitate ethical use of AI.
Lastly, continuous updates and improvements to AI systems are crucial for maintaining their effectiveness. However, these updates must be conducted ethically, ensuring that they do not compromise the integrity of the data or the fairness of the algorithms. As we embrace the potential of AI in enhancing disaster predictions, we must also be vigilant about these ethical considerations to build public trust and ensure responsible usage.
Frequently Asked Questions
- How does AI improve the accuracy of natural disaster predictions?
AI enhances prediction accuracy by analyzing vast amounts of data from various sources, like satellite imagery and historical records. By identifying patterns and trends, AI can forecast disasters more reliably than traditional methods.
- What types of data are used in AI disaster predictions?
AI relies on a diverse range of data, including satellite imagery, seismic data, and meteorological information. These datasets provide critical insights that help in making timely and accurate predictions.
- Can AI predict all types of natural disasters?
While AI has made significant strides in predicting events like hurricanes, earthquakes, and floods, its effectiveness can vary based on the type of disaster and the availability of quality data.
- What are the main benefits of using AI in disaster management?
The key benefits include improved prediction accuracy, faster response times, and better resource allocation. This leads to more effective disaster preparedness and recovery efforts, ultimately saving lives and reducing costs.
- What challenges does AI face in disaster prediction?
AI faces challenges such as data quality issues, algorithm biases, and the need for continuous updates. These factors can impact the reliability of predictions and the effectiveness of disaster response strategies.
- How can ethical considerations affect AI in disaster prediction?
Ethical considerations are crucial as they address data privacy, algorithm transparency, and accountability. Ensuring responsible AI usage is vital for maintaining public trust and effective disaster management.