The Growing Role of AI in Cybersecurity
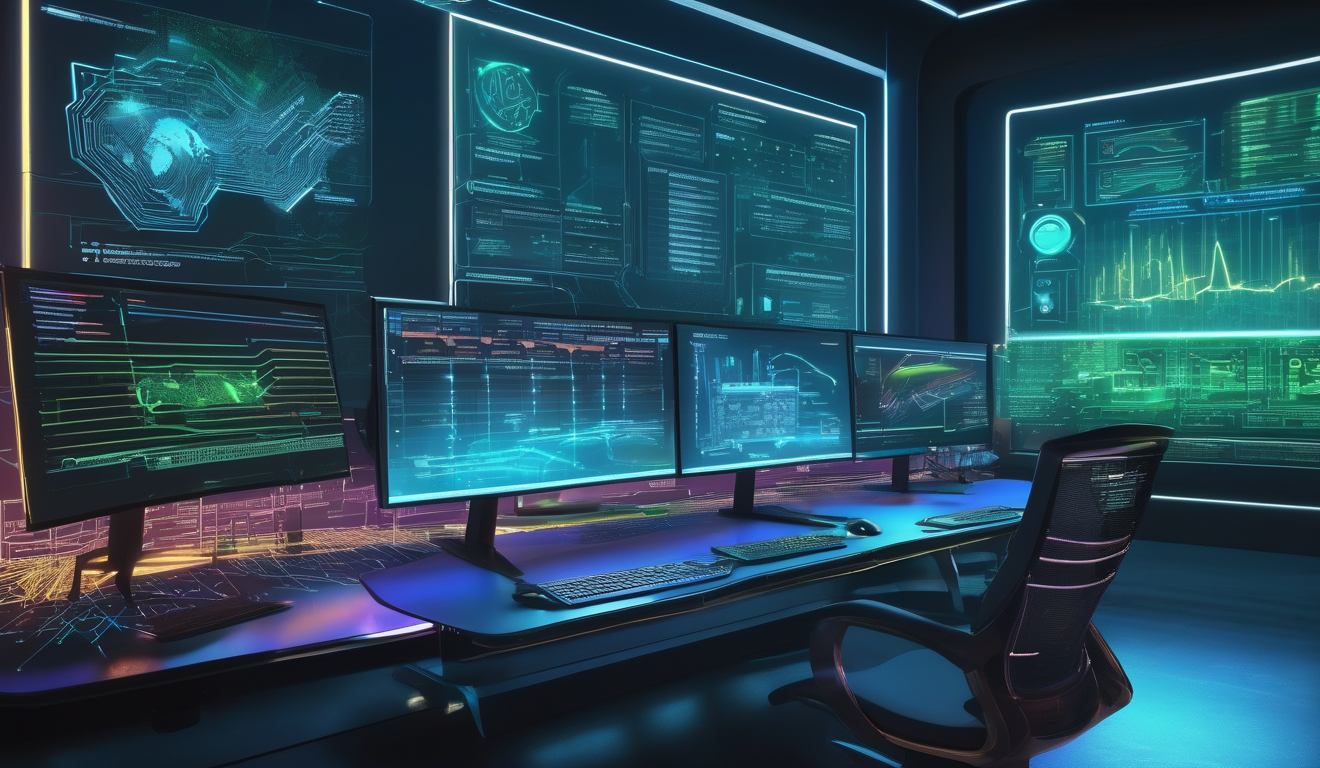
In today’s digital world, where everything is interconnected, the importance of cybersecurity has skyrocketed. With the rise of sophisticated cyber threats, organizations are increasingly turning to artificial intelligence (AI) to bolster their defenses. Imagine having a vigilant guard that never sleeps, constantly monitoring for intruders—that’s the essence of AI in cybersecurity. It’s not just about reacting to threats anymore; it’s about predicting and preventing them before they can cause harm.
AI is revolutionizing how we approach security challenges. By leveraging advanced algorithms and machine learning, AI systems can analyze enormous volumes of data in real-time. This capability allows them to identify patterns, detect anomalies, and flag potential risks that human analysts might overlook. Think of it as having a super-intelligent assistant that can sift through mountains of information in the blink of an eye!
Moreover, the adaptability of AI is a game changer. As cyber threats evolve, so do AI systems. They learn from past incidents and continuously improve their detection methods. This is crucial in a landscape where hackers are constantly developing new tactics. With AI, organizations can stay one step ahead, effectively transforming their cybersecurity posture from reactive to proactive.
But how does this all work? It boils down to two key concepts: machine learning and behavioral analytics. Machine learning algorithms allow systems to learn from historical data, adapting to new threats as they emerge. On the other hand, behavioral analytics focuses on understanding user behavior to spot any irregularities that might indicate a breach. Together, these technologies create a robust framework for identifying and mitigating risks.
As we delve deeper into the various applications of AI in cybersecurity, it becomes clear that its role is not just beneficial but essential. From automating incident response to enhancing security protocols, AI is paving the way for a more secure digital future. Are you ready to embrace the AI revolution in cybersecurity? The time to act is now, as the stakes have never been higher!
AI-Powered Threat Detection
In today’s digital age, the sheer volume of data generated every second is staggering. This is where artificial intelligence (AI) steps in, revolutionizing the way we approach threat detection. Imagine trying to find a needle in a haystack; that’s what cybersecurity professionals face without AI. By leveraging advanced algorithms, AI can sift through vast amounts of data in real-time, pinpointing anomalies that could signify a potential security breach.
AI’s ability to analyze data is akin to having a highly skilled detective who never sleeps. It constantly monitors network activity, identifying patterns and behaviors that deviate from the norm. This proactive approach enables organizations to detect threats before they escalate into full-blown attacks. In fact, studies show that companies using AI for threat detection can reduce response times by up to 40%, which is crucial in today’s fast-paced cyber landscape.
Moreover, AI can predict potential security breaches by analyzing historical data and recognizing trends. For instance, if a certain type of attack is detected frequently during specific times or events, AI can alert the security team to be on high alert during those periods. This predictive capability is like having a weather forecast for cyber threats, allowing organizations to prepare and fortify their defenses ahead of time.
To illustrate the impact of AI in threat detection, consider the following table that highlights key benefits:
Benefit | Description |
---|---|
Real-Time Monitoring | Constant analysis of network traffic to identify threats as they occur. |
Anomaly Detection | Identifies unusual patterns that may indicate a security breach. |
Predictive Alerts | Forecasts potential attacks based on historical data. |
In summary, AI-powered threat detection is not just an enhancement; it’s a game changer in the cybersecurity arena. With its ability to analyze data swiftly and accurately, organizations can stay one step ahead of cybercriminals. As the threats continue to evolve, embracing AI technology will be essential for maintaining robust security measures.
Automated Incident Response
In today’s digital landscape, the speed at which cyber threats emerge can be staggering. is becoming a game-changer for organizations striving to protect their sensitive data. Imagine a fire alarm that not only alerts you to danger but also calls the fire department and unlocks the doors for them. That’s the essence of automation in cybersecurity!
When a cyberattack occurs, every second counts. Traditional methods often lead to delays that can result in significant damage. However, with AI-driven solutions, organizations can react swiftly and efficiently. Automated systems can detect threats, assess their severity, and initiate predefined responses without human intervention. This not only minimizes potential damage but also allows security teams to focus on more complex tasks that require human insight.
For instance, consider a scenario where a suspicious login attempt is detected. An automated incident response system can instantly:
- Isolate the affected account
- Notify the security team
- Initiate a password reset
- Log the incident for further analysis
This level of automation ensures that potential breaches are contained before they escalate. Furthermore, the integration of machine learning algorithms allows these systems to continuously improve their response strategies. By analyzing past incidents, they can adapt and refine their actions, making future responses even more effective.
Moreover, the efficiency of automated incident response can significantly reduce recovery times. Organizations can bounce back from attacks more swiftly, ensuring business continuity and protecting their reputation. The cost savings associated with reduced downtime and damage control can be substantial, making automation not just a smart choice but a necessary one in today’s cyber threat environment.
In conclusion, as cyber threats become increasingly sophisticated, the need for automated incident response solutions will only grow. By leveraging AI, organizations can not only enhance their security posture but also foster a proactive approach to cybersecurity. Embracing this technology is not just about keeping up; it’s about staying ahead in the relentless race against cybercriminals.
Machine Learning Algorithms
When it comes to enhancing cybersecurity, are the unsung heroes working tirelessly behind the scenes. These algorithms are designed to analyze vast amounts of data, learning from past incidents to identify patterns and anomalies that could signal a potential threat. Imagine having a digital detective that never sleeps, constantly sifting through data to catch the bad guys before they even strike.
One of the key aspects of these algorithms is their ability to adapt. As cyber threats evolve, so do the methods used by machine learning systems. They can be classified into two main categories: supervised learning and unsupervised learning. Understanding these categories is crucial for organizations looking to implement effective cybersecurity measures.
Supervised learning involves training a model on a labeled dataset, where the algorithm learns to recognize patterns based on examples provided. For instance, if an organization feeds the model data about previous cyberattacks, it can learn to identify similar threats in the future. On the other hand, unsupervised learning allows the algorithm to explore data without any prior labels, identifying hidden patterns or anomalies that may not be immediately obvious.
Furthermore, the real-time analysis capability of machine learning algorithms significantly enhances threat detection. By continuously monitoring network traffic, these systems can flag unusual activities almost instantaneously. This is like having a security camera that not only records but also alerts you when something suspicious happens.
Incorporating machine learning into cybersecurity strategies not only improves detection rates but also reduces false positives. This means that organizations can focus their resources on genuine threats, rather than wasting time on benign anomalies. In a world where every second counts during a cyberattack, this efficiency can be the difference between a minor incident and a catastrophic breach.
In conclusion, machine learning algorithms are revolutionizing the way organizations approach cybersecurity. By leveraging these advanced technologies, businesses can stay one step ahead of cybercriminals, ensuring their data and systems remain secure. It’s like having a highly trained security team that learns and evolves with every challenge they face.
Supervised vs. Unsupervised Learning
When diving into the world of AI in cybersecurity, understanding the distinctions between supervised and unsupervised learning is crucial. These two approaches serve different purposes and can significantly impact how organizations train their cybersecurity models. Think of supervised learning as a teacher guiding students through a structured curriculum, while unsupervised learning is like a student exploring a new subject without any guidance. Both methods have their unique strengths and applications in the cybersecurity landscape.
In supervised learning, algorithms are trained on labeled datasets, which means they learn from examples that have both input data and the corresponding correct output. This approach is highly effective for tasks like threat classification and spam detection. For instance, a supervised learning model can be trained to recognize patterns of known cyber threats by analyzing historical data. The model is provided with examples of previous attacks, allowing it to predict and identify similar threats in real-time.
On the other hand, unsupervised learning deals with unlabeled data. Here, the algorithm tries to identify patterns and relationships within the data without prior knowledge of what to look for. This method is particularly valuable for detecting anomalies or unknown threats that haven’t been seen before. For example, if a user suddenly accesses sensitive files at an unusual hour, an unsupervised learning model can flag this behavior as suspicious, even if that specific threat was never encountered before.
To summarize, here’s a quick comparison:
Feature | Supervised Learning | Unsupervised Learning |
---|---|---|
Data Type | Labeled | Unlabeled |
Purpose | Predict outcomes | Discover patterns |
Example Use | Threat classification | Anomaly detection |
In conclusion, both supervised and unsupervised learning play vital roles in enhancing cybersecurity measures. By leveraging these techniques, organizations can better prepare for and respond to the ever-evolving landscape of cyber threats.
Real-Time Analysis
In today’s fast-paced digital landscape, has become a game changer for cybersecurity. Imagine trying to catch a thief in a crowded mall; the quicker you spot suspicious behavior, the better your chances of preventing a crime. This analogy perfectly illustrates the role of AI in monitoring network traffic. By analyzing data as it flows, AI systems can identify potential threats almost instantaneously, allowing organizations to act before damage occurs.
Real-time analysis leverages advanced algorithms to sift through enormous volumes of data, looking for patterns and anomalies that could indicate a security breach. This process isn’t just about speed; it’s about precision. For instance, AI can detect subtle deviations in user behavior, such as a sudden spike in data downloads or access attempts from unusual locations. These anomalies can serve as red flags, alerting security teams to investigate further.
Moreover, the integration of machine learning enhances the effectiveness of real-time analysis. As the AI system processes more data, it learns from past incidents, refining its ability to distinguish between normal activity and potential threats. This continual learning cycle enables organizations to stay one step ahead of cybercriminals, who are constantly evolving their tactics. In fact, studies have shown that organizations using AI-driven real-time analysis can reduce their incident response times significantly, often by as much as 50%.
To illustrate the impact of real-time analysis, consider the following table that highlights key benefits:
Benefit | Description |
---|---|
Immediate Threat Detection | Identifies potential breaches as they happen, allowing for swift action. |
Reduced Downtime | Minimizes the operational impact of cyberattacks by enabling faster recovery. |
Enhanced Security Posture | Improves overall defense mechanisms by continuously monitoring and adapting. |
In conclusion, the power of real-time analysis in cybersecurity cannot be overstated. It not only enhances threat detection but also empowers organizations to respond proactively to incidents. As cyber threats become more sophisticated, the need for such advanced analytical capabilities will only grow. Organizations that invest in real-time analysis will not only protect their assets but also gain a competitive edge in the digital arena.
Behavioral Analytics
In the ever-evolving landscape of cybersecurity, emerges as a powerful ally. This innovative approach leverages artificial intelligence to scrutinize user activities and identify patterns that may indicate potential threats. Imagine having a security guard who not only watches the door but also knows the habits of every individual who enters. Behavioral analytics does just that—it keeps an eye on user behavior, flagging any deviations from the norm that could signify a security breach.
One of the most compelling aspects of behavioral analytics is its ability to detect unusual user behavior. For instance, if an employee who usually accesses data during regular office hours suddenly logs in at midnight and downloads sensitive information, this anomaly could raise a red flag. By analyzing these behavioral patterns, organizations can pinpoint compromised accounts or insider threats before they escalate into full-blown incidents.
Moreover, behavioral analytics is not just about identifying risks; it’s also a proactive measure that helps organizations strengthen their defenses. By understanding how users typically interact with their systems, cybersecurity teams can develop tailored security protocols that adapt to the unique behaviors of their workforce. This customization ensures that security measures are not only effective but also user-friendly, reducing the likelihood of employee pushback.
To illustrate the impact of behavioral analytics, consider the following table that outlines its key benefits:
Benefit | Description |
---|---|
Early Threat Detection | Identifies anomalies in user behavior that may indicate a security breach. |
Enhanced Security Protocols | Allows for the development of customized security measures based on user habits. |
Reduced False Positives | Minimizes unnecessary alerts by focusing on significant behavioral changes. |
In conclusion, behavioral analytics represents a significant leap forward in cybersecurity strategies. By harnessing the power of AI to monitor and analyze user behavior, organizations can not only detect threats more effectively but also build a more resilient security posture. As cyber threats continue to grow in sophistication, embracing behavioral analytics will be crucial for any organization looking to stay one step ahead.
Enhanced Security Protocols
In today’s digital age, where cyber threats are evolving at a breakneck speed, are not just a luxury; they are a necessity. Organizations are increasingly turning to artificial intelligence to fortify their defenses against these sophisticated attacks. By integrating AI into their security frameworks, companies can create a more resilient and responsive security posture that adapts to the ever-changing landscape of cyber threats.
AI plays a pivotal role in developing robust security protocols that can withstand various attack vectors. One of the significant advantages of using AI is its ability to learn and improve over time. This continuous learning process allows organizations to stay ahead of potential threats, making it easier to implement proactive measures. For instance, AI can analyze historical data and current threat intelligence to identify patterns that may indicate an impending attack.
Moreover, AI-driven security protocols can facilitate real-time threat detection and response. Imagine having a security system that not only alerts you to a breach but also takes immediate action to mitigate the damage. With AI, this scenario is becoming a reality. By automating the response process, organizations can significantly reduce the time it takes to identify and neutralize threats, which is crucial in minimizing potential damage.
Another essential aspect of enhanced security protocols is predictive analytics. By leveraging AI’s predictive capabilities, organizations can forecast potential vulnerabilities and strengthen their defenses before an attack occurs. This proactive approach not only saves time and resources but also instills confidence in stakeholders that the organization is prepared for any eventuality.
Furthermore, AI-driven risk assessment tools provide valuable insights into an organization’s security posture. These tools can help identify weak points in the security infrastructure, enabling organizations to prioritize their resources and strategies effectively. For example, a comprehensive risk assessment may reveal that certain areas are more susceptible to attacks, allowing for targeted investments in security measures.
In conclusion, the integration of AI into enhanced security protocols is revolutionizing the way organizations approach cybersecurity. By adopting these advanced technologies, companies can not only protect themselves against current threats but also prepare for future challenges in the digital landscape.
Predictive Analytics
In today’s fast-paced digital world, stands out as a game-changer in cybersecurity. Imagine having a crystal ball that not only shows you the current state of your network but also predicts where vulnerabilities might arise in the future. That’s precisely what predictive analytics offers! By analyzing historical data and recognizing patterns, organizations can foresee potential threats before they escalate into full-blown attacks.
So, how does this magic happen? Well, it all starts with data. The more data you feed into your predictive models, the more accurate your forecasts become. Think of it like training a puppy; the more you teach it, the better it performs. Predictive analytics uses complex algorithms to sift through mountains of data, identifying trends that might go unnoticed by the human eye. This allows cybersecurity teams to focus their efforts on the most pressing threats, rather than spreading themselves thin across every potential issue.
Moreover, predictive analytics isn’t just about spotting threats; it’s also about strengthening defenses. Organizations can proactively implement security measures based on the insights gained from these predictions. For instance, if a particular type of attack is forecasted to increase, companies can bolster their defenses in that area before the attack even occurs. This proactive approach can save time, money, and a lot of headaches in the long run.
Here’s a quick overview of the benefits of predictive analytics in cybersecurity:
- Proactive Threat Management: Organizations can address vulnerabilities before they are exploited.
- Resource Optimization: By focusing on high-risk areas, companies can allocate resources more effectively.
- Improved Incident Response: Faster identification of threats leads to quicker responses, minimizing damage.
In conclusion, as cyber threats become more sophisticated, the need for advanced tools like predictive analytics grows ever more critical. By leveraging these insights, organizations can not only defend against current threats but also anticipate and prepare for future challenges. In the world of cybersecurity, being one step ahead is not just an advantage; it’s a necessity.
Risk Assessment
In the ever-evolving landscape of cybersecurity, stands out as a critical component. It’s like having a weather forecast for your digital environment—identifying potential storms before they wreak havoc. AI-driven risk assessment tools provide organizations with invaluable insights into their security posture. By analyzing data from various sources, these tools can pinpoint vulnerabilities that might otherwise go unnoticed.
Imagine trying to protect your home without knowing where the weak spots are. That’s exactly what a company faces without a comprehensive risk assessment. With AI, organizations can not only identify these weak spots but also prioritize them based on the level of risk they pose. This proactive approach allows businesses to allocate resources more effectively, ensuring that the most critical vulnerabilities are addressed first.
Moreover, AI enhances the risk assessment process by incorporating predictive analytics. This means organizations can forecast potential threats based on historical data and current trends. For instance, if a particular type of cyberattack has been on the rise in the industry, AI can alert organizations to bolster defenses against similar attacks before they happen. This forward-thinking strategy is akin to a chess player anticipating their opponent’s moves, allowing for a more strategic defense.
To illustrate the benefits of AI in risk assessment, consider the following table that outlines the key advantages:
Advantage | Description |
---|---|
Proactive Threat Identification | AI can predict and identify potential threats before they materialize. |
Resource Optimization | Helps prioritize security measures based on risk levels, ensuring efficient use of resources. |
Continuous Learning | AI systems learn from new data, adapting to emerging threats dynamically. |
In conclusion, integrating AI into risk assessment not only enhances an organization’s ability to identify and mitigate threats but also fosters a culture of security awareness. By understanding their vulnerabilities and acting on them, companies can build a robust defense mechanism that stands strong against the relentless tide of cyber threats.
Frequently Asked Questions
- How does AI enhance threat detection in cybersecurity?
AI enhances threat detection by analyzing large volumes of data in real-time, spotting anomalies, and predicting potential security breaches before they happen. It’s like having a super-smart assistant that never sleeps, constantly on the lookout for anything suspicious!
- What role do machine learning algorithms play in cybersecurity?
Machine learning algorithms are crucial as they allow systems to learn from past data and adapt to new threats. Imagine teaching a dog new tricks; the more you train it, the better it gets at recognizing commands. Similarly, these algorithms improve over time, becoming more effective at identifying and mitigating risks.
- What is the difference between supervised and unsupervised learning?
Supervised learning involves training a model on labeled data, meaning it knows what to look for. Unsupervised learning, on the other hand, works with unlabeled data, trying to find patterns on its own. Think of it like having a map versus exploring a new city without one!
- How does AI streamline incident response?
AI automates many aspects of incident response, allowing organizations to react quickly to threats, minimizing damage, and speeding up recovery. It’s like having a fire alarm that not only alerts you but also starts extinguishing the fire before it spreads!
- What are predictive analytics in the context of cybersecurity?
Predictive analytics uses historical data to forecast potential vulnerabilities, helping organizations bolster their defenses before attacks occur. Imagine having a crystal ball that shows you where danger might come from, allowing you to prepare in advance!